Ethical Dilemmas of Generative AI A Deep Dive
Ethical dilemmas of generative AI are rapidly emerging as a critical concern. From the potential for bias in training data to the complexities of intellectual property, the technology raises complex questions about responsibility, accountability, and the future of human creativity. This exploration delves into the multifaceted challenges posed by generative AI, examining its impact on society, the economy, and individual rights.
This article examines the key ethical concerns surrounding generative AI, including bias, misinformation, job displacement, privacy, and the difficult task of assigning responsibility for AI-generated content. By exploring these issues, we can begin to understand the potential benefits and drawbacks of this transformative technology and work towards developing ethical guidelines for its responsible development and deployment.
Bias and Fairness in Generative AI
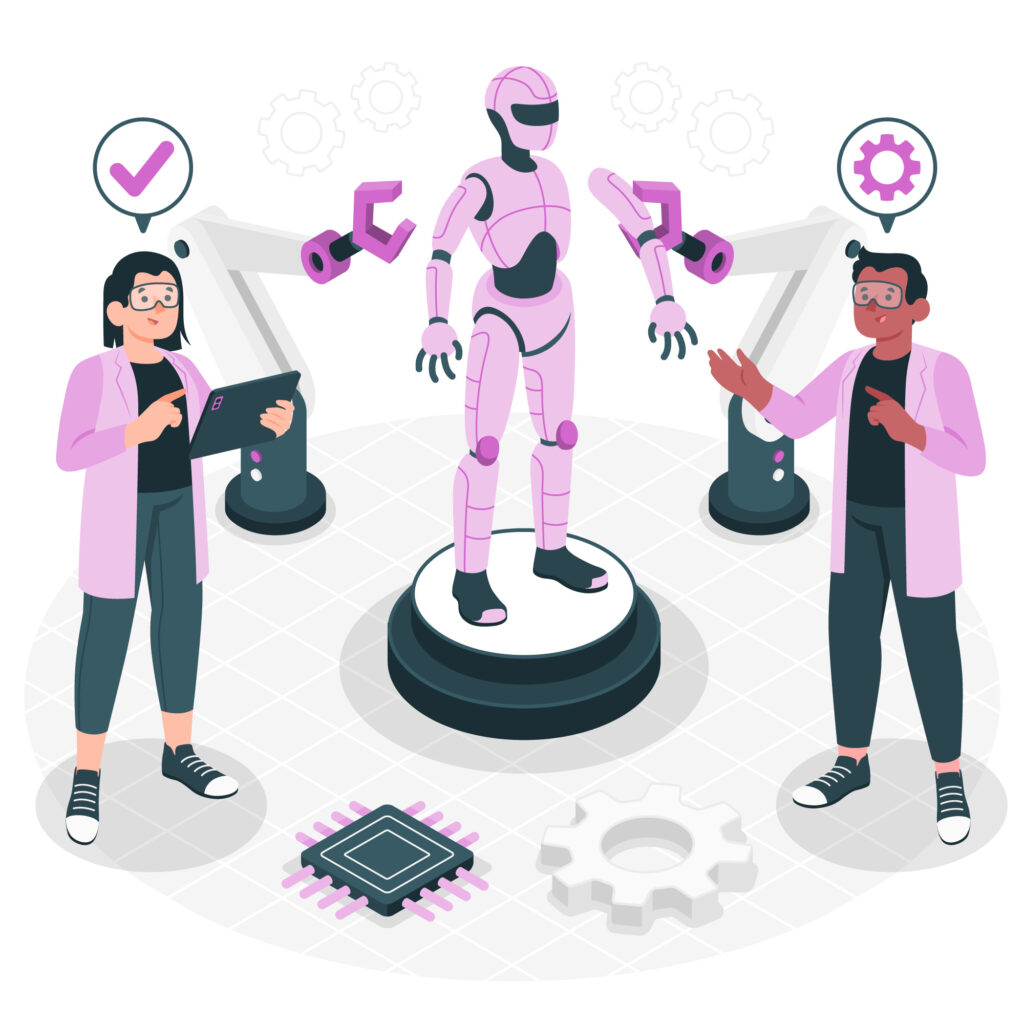
Generative AI models, capable of creating novel content, are revolutionizing various fields. However, these models are trained on vast datasets, which can reflect existing societal biases. These biases can then be amplified and reproduced in the generated content, leading to potentially harmful or discriminatory outcomes. Addressing these biases is crucial for ensuring fairness and ethical use of generative AI.The potential for bias in generative AI systems stems from the very data they are trained on.
If the training data reflects societal stereotypes or prejudices, the model will learn and perpetuate these biases, potentially leading to skewed or harmful outputs. Understanding and mitigating these biases is paramount to responsible AI development and deployment.
Generative AI is raising some serious ethical questions, like who owns the rights to the content it creates. Thinking about the passing of a legendary actress like Linda Lavin, Tony Award-winning Broadway star and star of the sitcom “Alice,” Linda Lavin , reminds us that creativity and artistry have always faced ethical challenges. It makes you ponder the impact of AI on the future of human expression and the creative industries.
Potential Biases in Training Datasets
Generative AI models are trained on massive datasets, often encompassing text, images, and audio. These datasets, if not carefully curated, can inadvertently reflect existing societal biases. For instance, a dataset used to train a text-generation model might disproportionately feature male characters in leadership roles or portray certain ethnic groups in stereotypical ways. Similarly, an image dataset used to train a style transfer model might contain images that reinforce gender or racial stereotypes.
These biases are then encoded within the model’s structure, leading to the generation of potentially unfair or harmful content.
Manifestation of Biases in Generated Content
The biases embedded in training data can manifest in various ways within the generated content. A text-generation model might produce biased descriptions of historical figures, perpetuating inaccuracies and misrepresentations. An image-generation model might consistently produce images of people of a specific gender or race in stereotypical roles. These manifestations can have significant real-world consequences, contributing to the perpetuation of societal inequalities.
For example, if a model consistently portrays women in subservient roles, it reinforces existing gender stereotypes, limiting the potential for progress in equality.
Methods for Detecting and Mitigating Bias
Identifying and mitigating biases in generative AI systems is a complex but crucial task. Various techniques can be employed to detect and reduce the impact of biases.
- Data Analysis and Auditing: Thoroughly examining the training data for potential biases is essential. Analyzing the distribution of different groups, genders, and ethnicities within the dataset can reveal patterns that might reflect bias. Further analysis should examine the presence of stereotypes and harmful language within the data.
- Bias Detection Algorithms: Specialized algorithms are designed to identify biases in datasets and models. These algorithms can analyze the data and flag patterns that suggest bias, prompting further investigation and data cleaning.
- Human Evaluation and Feedback: Human review of generated content is essential for detecting biases that might be missed by automated methods. Feedback from diverse groups of individuals can help identify instances of unfair or harmful content.
- Data Augmentation and Cleaning: Actively working to balance the representation of different groups in the dataset is critical. Methods like oversampling underrepresented groups or removing data containing biases can improve the model’s fairness.
Bias Detection Techniques Comparison
Technique | Description | Strengths | Weaknesses |
---|---|---|---|
Data Analysis and Auditing | Manual or automated examination of the training data for patterns of bias. | Simple to implement in initial stages; helps understand the nature of bias. | Can be time-consuming; may miss subtle biases; subjective interpretation possible. |
Bias Detection Algorithms | Algorithms specifically designed to identify biased patterns in data. | Objective and efficient in detecting specific biases. | May not capture all forms of bias; can be computationally expensive. |
Human Evaluation and Feedback | Human review and feedback on generated content to identify biases. | Provides a crucial human perspective on the potential harm of generated content; can identify subtle biases. | Subjective and time-consuming; potentially inconsistent across evaluators. |
Data Augmentation and Cleaning | Actively working to balance the representation of different groups in the dataset. | Improves the fairness of the model; directly addresses the root cause of bias. | Can be challenging to determine the appropriate balance; may introduce new biases. |
Intellectual Property and Ownership
The rise of generative AI has introduced unprecedented complexities to the realm of intellectual property. Determining ownership of content created by these systems is challenging, prompting legal and ethical debates worldwide. The traditional models of copyright, often centered around human authorship, are struggling to adapt to the novel nature of AI-generated works. This necessitates a critical examination of existing legal frameworks and potential conflicts between human creators and AI systems.The ownership of intellectual property generated by AI systems is a significant legal and ethical concern.
Existing copyright laws, primarily focused on human authorship, often struggle to accommodate the creative output of AI. This creates a void where the legal status of AI-generated content is unclear, leading to potential conflicts between the rights of human creators and the rights of the AI systems themselves. This intricate situation requires careful consideration and potential revisions to existing frameworks to accommodate the unique characteristics of AI-generated works.
Copyright Scenarios Involving AI-Generated Art
Determining copyright ownership for AI-generated content necessitates a nuanced approach, considering various scenarios and the roles of different parties involved. The table below Artikels potential copyright scenarios, highlighting the complexities of this emerging field.
Scenario | Copyright Holder | Reasoning |
---|---|---|
Example 1: AI trained on a dataset of existing artwork creates a new piece. | Potentially the owner of the dataset, or the developer of the AI. | The AI is a tool used to process and transform the dataset. The question becomes whether the AI significantly altered the existing data or merely recombined it in a new form. If the output is a mere rearrangement of existing elements, the ownership might be tied to the original copyright holders of the dataset. If it significantly transforms the elements, the argument for the AI’s developer or the dataset owner becoming the copyright holder strengthens. |
Example 2: A human prompts an AI to generate an image, specifying artistic style and subject matter. | The human prompt provider. | The human input significantly dictates the creative output. The AI acts as a tool, processing the prompt. The prompt itself, therefore, can be considered the creative driving force, establishing the human’s claim to ownership. |
Example 3: An AI generates a unique piece of music based on its own internal algorithms, with no human input. | The AI developer or the company that owns the AI. | The AI, in this instance, is the primary creative agent. Ownership is likely vested in the entity that developed and owns the AI system. This is similar to ownership of a creative product by a software program, for example. |
Example 4: An AI is used to create a novel that was heavily edited and developed by a human author. | The human author. | The AI’s role is primarily as a tool for generating text. The human author’s significant editing and development of the final product establishes their claim to ownership of the copyright. |
Legal Frameworks Regarding Ownership, Ethical dilemmas of generative ai
Different jurisdictions are developing various legal frameworks to address the ownership of AI-generated works. Some countries have existing copyright laws that could potentially be applied, while others are exploring new approaches. These varying legal interpretations create challenges in establishing consistent global standards for AI-generated content. For example, some legal frameworks prioritize the role of the human creator, while others may focus on the AI’s contribution to the creative process.
Potential Conflicts Between Human Creators and AI Systems
The interplay between human creators and AI systems raises the prospect of potential conflicts over intellectual property rights. These conflicts can arise when an AI system generates content that closely resembles or is a derivative of existing works created by humans. Such conflicts often hinge on the degree of originality and transformation introduced by the AI system.
Misinformation and Disinformation
Generative AI, while offering exciting possibilities, presents a significant challenge in the realm of information accuracy. The ease with which AI can create realistic, yet fabricated content raises concerns about the spread of misinformation and disinformation. This poses a serious threat to public trust, democratic processes, and even individual safety. Identifying and combating this threat requires a multifaceted approach that combines technical solutions with public awareness campaigns.The potential for AI to generate convincingly false content, ranging from simple fabricated news articles to complex, multimedia forgeries, is a major concern.
This capability necessitates a proactive approach to ensure the accuracy of information shared online and across different platforms.
Examples of AI-Generated Misinformation
Generative AI can create convincingly realistic text, images, and audio, making it difficult to discern genuine content from fabricated material. For example, AI-generated articles mimicking established news outlets could spread false information about political events or scientific discoveries. Deepfakes, synthetic videos of individuals making false statements, are another potent method of misinformation. Similarly, AI-generated audio can be used to impersonate individuals, spreading false rumours or propaganda.
This capability is not limited to simple text or images, as AI can create intricate simulations of complex events or processes, making it hard to distinguish fact from fiction.
Methods to Identify AI-Generated Misinformation
Identifying AI-generated content requires sophisticated techniques. The characteristics of AI-generated content often differ from human-created content, making it possible to distinguish between them. A variety of methods are being developed to detect AI-generated content.
Potential Societal Impact of AI-Generated Disinformation
The widespread adoption of AI-generated misinformation could have profound societal impacts. Loss of public trust in information sources could erode democratic processes and lead to political instability. Misinformation campaigns targeting individuals or groups could lead to social unrest or even violence. In the economic realm, financial markets could be manipulated by AI-generated false information, resulting in significant losses.
The impact could be further amplified by the speed and scale at which AI-generated content can be disseminated.
Methods to Detect AI-Generated Text
Method | Description | Accuracy | Limitations |
---|---|---|---|
Statistical Analysis | Identifying patterns and anomalies in the text’s linguistic structure, such as unusual word choices or sentence structures. | Moderate | Can be easily bypassed by advanced AI models; may not detect subtle manipulation. |
Neural Network Detection | Training a neural network to recognize characteristics of AI-generated text, using a dataset of known AI-generated and human-written text. | High | Requires a large and well-labeled dataset; can be expensive and time-consuming to develop. |
Linguistic Analysis | Examining the grammatical structure, style, and vocabulary of the text to identify potential inconsistencies. | Moderate | Effectiveness depends on the sophistication of the language model used to generate the text; may not catch complex forgeries. |
Contextual Analysis | Checking the source and context of the information to determine if it aligns with established facts and information. | High | Requires thorough research and verification; effectiveness depends on the reliability of the sources being checked. |
Job Displacement and Economic Impacts: Ethical Dilemmas Of Generative Ai
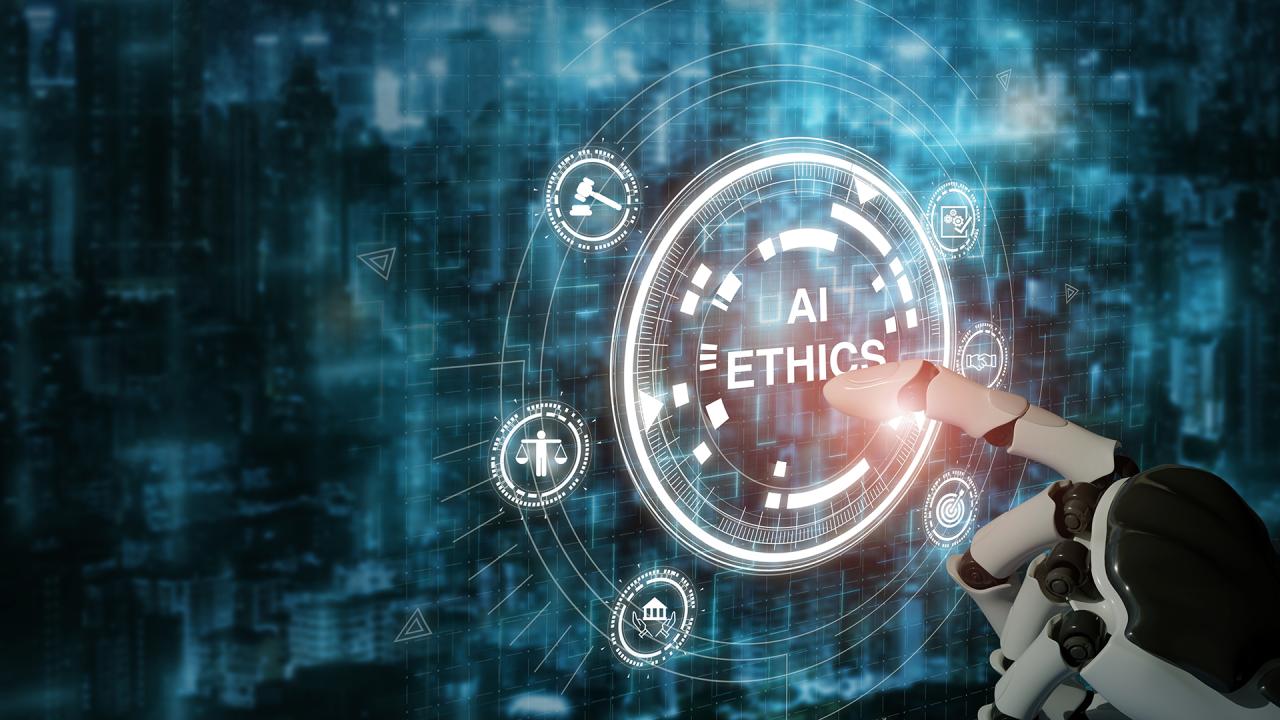
Generative AI’s potential to automate tasks previously performed by humans is undeniable, raising profound questions about its impact on employment markets. This automation, while promising efficiency gains in certain sectors, also presents the possibility of widespread job displacement and requires careful consideration of the associated economic ramifications. Understanding both the potential benefits and drawbacks is crucial for navigating this transformative technology responsibly.The rapid evolution of generative AI tools presents a complex interplay of positive and negative consequences for the job market.
Automation driven by AI has the potential to boost productivity and efficiency across various industries, leading to increased output and potentially lower costs for consumers. However, the same automation can lead to significant job displacement as AI-powered systems take over tasks currently performed by human workers. The key lies in adapting to this change and focusing on reskilling and upskilling the workforce to prepare for the evolving job landscape.
Potential for Generative AI to Automate Tasks
Generative AI’s ability to mimic human creativity and perform complex tasks is constantly expanding. This capability extends to various domains, from writing articles and composing music to designing products and even coding software. Consequently, many roles previously requiring human expertise could potentially be automated. Examples include data entry, customer service interactions, and even certain types of legal and administrative work.
Possible Consequences for Employment Markets
The automation potential of generative AI raises concerns about potential job losses. Sectors heavily reliant on routine tasks are particularly vulnerable to AI-driven automation. This could lead to increased unemployment and inequality, necessitating proactive measures to mitigate these risks. Strategies for retraining and upskilling workers are critical to navigate this transition effectively.
Potential Benefits and Drawbacks of Generative AI in the Workplace
Generative AI offers numerous potential benefits for businesses, such as increased efficiency, reduced operational costs, and improved decision-making processes. These benefits stem from AI’s ability to process large amounts of data, identify patterns, and automate repetitive tasks. However, these benefits come with potential drawbacks. One significant drawback is the displacement of human workers, requiring adjustments to the workforce and potential economic disruption.
Impact on Different Roles
The potential impact of generative AI on various roles is multifaceted. A table outlining potential impacts and mitigation strategies can provide a framework for understanding this dynamic.
Role | Potential Impact | Mitigation Strategies |
---|---|---|
Data Entry Clerks | High probability of automation, leading to job displacement. | Upskilling in data analysis, AI-related fields, or roles that leverage AI for increased efficiency. |
Customer Service Representatives | Automation of routine inquiries through AI chatbots. | Training in advanced communication skills, problem-solving, and emotional intelligence to complement AI-driven systems. |
Writers and Content Creators | Generative AI can produce initial drafts and content. | Focus on unique insights, analysis, and storytelling to distinguish human-created content. |
Software Developers | AI tools can automate code generation, leading to potential productivity gains. | Focus on higher-level software design, problem-solving, and project management roles. |
Graphic Designers | AI tools can generate images and designs. | Focus on creativity, design direction, and conceptualization to differentiate human-designed content. |
Privacy and Data Security
Generative AI models, like large language models, are trained on massive datasets. Understanding the role of data in their development is crucial for appreciating the potential risks to user privacy. These models learn patterns and relationships from vast quantities of text, code, and other data, which significantly impacts their ability to generate human-like text and perform various tasks.
The sheer volume of data involved raises concerns about the potential misuse of personal information.The training process often involves using publicly available data, but this can also include user-generated content, personal information, and sensitive data. This raises serious concerns about the potential for unauthorized access to personal information and its misuse. The potential for data breaches and the use of this data for malicious purposes requires careful consideration and robust safeguards.
Role of Data in Training Generative AI Models
Generative AI models, in their training phase, are fed vast amounts of data, which can include text, images, code, and more. This data is crucial for the models to learn patterns, relationships, and structures within the information. The models analyze this data to identify statistical correlations and construct intricate patterns that allow them to generate new content.
Potential Risks Related to User Data Privacy
The use of personal data in training generative AI models presents several privacy risks. Users may not be aware of how their data is being used, or what safeguards are in place to protect their privacy. Furthermore, the sheer volume of data and the complexity of the models can make it challenging to trace the origin of specific pieces of information.
Generative AI’s ethical pitfalls are complex, ranging from potential bias in generated content to concerns about job displacement. It’s fascinating to consider how these issues intertwine with broader societal trends, like the recent news surrounding some US companies scaling back on diversity initiatives, as detailed in which US companies are pulling back on diversity initiatives. Ultimately, these parallel trends highlight the need for careful consideration of the ethical implications of emerging technologies, and how they might exacerbate existing inequalities.
This raises concerns about potential biases in the generated output.
Generative AI is raising some serious ethical questions, like who owns the rights to the content it creates. Elon Musk’s involvement in European politics, particularly his views on AI regulation, elon musk europe politics , might offer some interesting insights into how to navigate these complex issues. Ultimately, finding a balance between innovation and ethical considerations is key for responsible AI development.
Examples of How Generative AI Could Violate Privacy
Generative AI models can be used to create realistic fake profiles, impersonate individuals, or reconstruct private data. For example, a model trained on social media posts could generate realistic text messages that mimic the style of a user. These text messages could be used to deceive or defraud the user. Another example involves generating convincing fake images or videos of individuals, which could be used for identity theft or harassment.
Moreover, sensitive information, such as medical records or financial data, might be inadvertently included in the training data, exposing individuals to risks of unauthorized disclosure or misuse.
Ways User Data is Collected and Used by AI
The following table illustrates the various ways user data is collected and used by generative AI models. Understanding these methods is critical for appreciating the privacy implications.
Collection Method | Usage | Potential Risks |
---|---|---|
Social media activity | Training on user posts, comments, and interactions. | Exposure of personal opinions, relationships, and locations. |
Search engine queries | Training on user search history, revealing preferences and interests. | Potential for targeted advertising and manipulation. |
Online purchases | Training on transaction details, revealing financial information and shopping habits. | Risk of financial fraud and unauthorized access to accounts. |
Device usage data | Training on usage patterns and device information. | Potential exposure of location, activities, and personal preferences. |
Responsibility and Accountability
Navigating the ethical landscape of generative AI necessitates a profound examination of responsibility and accountability. The ability of these systems to generate human-like text, images, and code presents unprecedented challenges in determining who is accountable when something created by AI causes harm. This is a complex issue with significant implications for individuals, organizations, and society as a whole.The lack of a clear chain of responsibility for harmful outputs from generative AI models is a significant concern.
Determining culpability becomes convoluted when considering the various actors involved – the AI developers, the users who prompt the models, and the platforms that host and distribute the outputs. Who bears the responsibility for the misuse of generated content, especially when it promotes misinformation, incites violence, or infringes on intellectual property rights?
Difficulty in Assigning Responsibility
Establishing clear lines of responsibility for AI-generated content is extremely challenging. Current legal frameworks often struggle to adapt to the unique characteristics of AI systems. Existing liability models, typically focused on human actions, are ill-equipped to address the complex interplay of human input and AI output. The lack of transparency in the AI decision-making process further complicates the issue, making it difficult to trace the origin of harmful actions.
Potential Legal Frameworks for Addressing Liability
Several potential legal frameworks could help address liability in AI-related incidents. These frameworks might include:
- Strict Liability: Holding developers strictly liable for harm caused by their AI systems, regardless of intent or negligence. This approach emphasizes the potential for significant harm from AI systems and aims to deter developers from creating dangerous systems. However, it might stifle innovation due to the high risk of liability.
- Negligence-Based Liability: Establishing liability based on the developers’ negligence in designing, implementing, or deploying the AI system. This approach requires demonstrating a lack of due care, which can be difficult to prove in the context of rapidly evolving AI technology.
- Comparative Responsibility: Distributing liability among the various actors involved in the creation and use of AI-generated content. This approach could factor in the degree of contribution from each actor. This would necessitate sophisticated frameworks for assessing the relative contributions.
Importance of Ethical Guidelines
Ethical guidelines are crucial for the responsible development and deployment of generative AI. These guidelines should address issues such as bias mitigation, transparency in AI decision-making, and responsible use of AI outputs. Developers must prioritize safety and well-being and strive for ethical outcomes.
- Transparency and Explainability: AI models should be designed with transparency and explainability in mind. This will allow for greater scrutiny of the decision-making processes, potentially reducing the likelihood of unintended or harmful outputs.
- Bias Mitigation: Explicit efforts must be made to minimize biases present in the training data and algorithms. This involves rigorous data analysis and algorithmic design to prevent the amplification of existing societal biases.
- Accountability Mechanisms: Robust accountability mechanisms are essential for addressing harmful outputs. These mechanisms should be integrated into the development and deployment processes, allowing for rapid responses to incidents and the implementation of corrective measures.
Challenges and Potential Solutions
Challenge | Potential Solution |
---|---|
Determining the responsibility for AI-generated misinformation | Implementing fact-checking and verification tools for AI-generated content. Establishing clear guidelines for the use of AI in news generation and content creation. |
Addressing the potential for AI-generated content to be used for malicious purposes | Developing systems to detect and mitigate the risks associated with malicious use of AI-generated content. This includes developing AI-based detection systems and implementing stricter regulations on AI development and use. |
Transparency and Explainability
Generative AI models, while powerful, often operate as “black boxes.” Understanding how these models arrive at their outputs is crucial for building trust and ensuring responsible use. This lack of transparency raises concerns about bias, fairness, and potential misuse. This section delves into the limitations of current generative AI model understanding and explores strategies to improve their explainability.The opacity of generative AI models poses significant challenges.
These models, trained on vast datasets, often learn complex relationships without explicitly representing the underlying logic. Predicting their outputs based on input features becomes difficult, making it challenging to identify potential biases or errors. This lack of transparency hinders the ability to verify the reliability and trustworthiness of generated content.
Limitations in Understanding Generative AI Outputs
Generative AI models, especially those based on deep learning architectures like transformers, operate in a complex multi-layered manner. Their internal workings are not always easily interpretable. The process of generating text, images, or code involves intricate interactions between numerous parameters and layers. These models can exhibit emergent behaviors, producing outputs that are surprising even to their developers.
Furthermore, understanding the specific factors influencing the output quality or accuracy is often difficult, particularly in more complex models. For instance, a large language model might generate a coherent and grammatically correct response, but it might be difficult to pinpoint the exact reasons why it selected a particular word or phrase from its training data.
Importance of Transparency in AI Systems
Transparency in AI systems is essential for building trust and accountability. When users understand how an AI system arrives at its decisions, they can better evaluate its reliability and fairness. Transparent systems are more amenable to audits and corrections, mitigating the risk of bias and errors. This understanding empowers users to identify potential biases, evaluate the validity of outputs, and adapt their interactions accordingly.
Enhancing the Explainability of Generative AI Models
Several techniques can enhance the explainability of generative AI models. These methods focus on providing insights into the model’s decision-making process. One approach involves visualizing the internal representations of the model, allowing developers to identify patterns and relationships. Another approach involves developing simpler, more interpretable models that mimic the behavior of complex generative AI models. For example, researchers are exploring techniques that break down complex models into smaller, more understandable components.
Furthermore, the use of attention mechanisms in transformer models allows us to see which parts of the input are most influential in the output, providing a degree of explainability.
Different Levels of Transparency in Generative AI
Level | Description | Implementation |
---|---|---|
Limited Transparency | The model’s internal workings are largely opaque. Output is produced without significant explanation. | Current state-of-the-art models often fall into this category. |
Partial Transparency | Some insight into the model’s decision-making process is available. For instance, attention mechanisms can highlight influential input elements. | Models incorporating attention mechanisms or simpler surrogate models can provide a degree of partial transparency. |
High Transparency | Detailed explanations of the model’s reasoning are provided. The model’s output is accompanied by justifications. | Future research and development are needed to achieve high transparency. Developing models with explainable components and interpretable representations would be crucial. |
End of Discussion
In conclusion, the ethical dilemmas posed by generative AI are substantial and multifaceted. From the algorithmic biases inherent in training data to the potential for widespread misinformation, the technology demands careful consideration of its societal impact. Addressing these concerns requires a collaborative effort involving researchers, policymakers, and the public to ensure that generative AI is developed and used responsibly, benefiting humanity while mitigating potential harms.