How to Download Instagram Data Using Python
How to download Instagram data using Python? This comprehensive guide dives into the world of extracting Instagram data programmatically. We’ll explore the ins and outs of using Python libraries, navigating the Instagram API, and extracting various data types like posts, comments, and even stories. Understanding the legal and ethical implications is crucial, and we’ll address those as well.
Get ready to unlock the power of Instagram data with Python!
From basic installation to advanced techniques, this guide covers every step, ensuring you can extract Instagram data efficiently and effectively. We’ll break down the process into manageable chunks, providing clear explanations and practical examples along the way. We’ll also delve into how to handle potential issues like API rate limits and dynamic content, ensuring your scraping operations run smoothly.
Introduction to Instagram Data Extraction
Instagram, a ubiquitous social media platform, offers a wealth of user-generated content. Programmatically accessing this data using Python unlocks valuable insights and opportunities for analysis, marketing, and research. From understanding user engagement patterns to identifying trending topics, the potential applications are vast. This exploration delves into the techniques, considerations, and ethical implications of extracting Instagram data.
Importance and Potential Applications
Extracting Instagram data programmatically allows for a deeper understanding of user behavior and trends. Businesses can leverage this data to refine marketing strategies, understand audience preferences, and tailor content to resonate with their target demographics. Researchers can use it to study social phenomena, analyze public opinion, and track the spread of information. Academic studies, market research, and personal projects can all benefit from the ability to access and analyze Instagram data.
Legal Considerations and Ethical Implications
Accessing Instagram data requires strict adherence to Instagram’s terms of service and relevant data privacy regulations. Collecting and using user data ethically is paramount. Respecting user privacy and obtaining explicit consent when necessary are crucial ethical considerations. Unauthorized data collection is a violation of these principles and can lead to severe legal repercussions. Instagram’s API and developer policies Artikel the permissible uses of data.
Furthermore, data protection regulations like GDPR (General Data Protection Regulation) must be considered when handling collected data.
Types of Extractable Data
The following table Artikels the types of data that can be extracted from Instagram using Python, along with some example use cases. The availability and specifics of data extraction are subject to change based on Instagram’s API and policies.
Data Type | Description | Example Use Cases |
---|---|---|
Posts | Content of user posts, including images, videos, captions, and associated metadata. | Tracking trending topics, analyzing content performance, identifying brand mentions. |
Comments | User comments on posts, including the text and author information. | Analyzing public sentiment towards a product, monitoring brand reputation, understanding community discussions. |
Followers | List of users who follow a particular account. | Identifying influential accounts, assessing account reach, understanding community growth. |
Interactions | Likes, shares, and other user interactions with posts and accounts. | Analyzing engagement rates, understanding content preferences, monitoring campaign effectiveness. |
Account Information | Details about Instagram accounts, such as bio, profile picture, and public information. | Conducting market research, identifying competitors, building comprehensive profiles of accounts. |
Python Libraries for Instagram Data Extraction
Extracting data from social media platforms like Instagram requires robust tools and libraries. Python, with its versatile ecosystem, offers several powerful options for this task. This section delves into popular Python libraries, highlighting their strengths and weaknesses in the context of Instagram data scraping.
Several Python libraries provide the functionality needed to retrieve Instagram data. Understanding their specific capabilities and limitations is crucial for effective data extraction. This discussion will focus on the key libraries, demonstrating their installation and use, and comparing their strengths and weaknesses for Instagram scraping.
Popular Python Libraries
Several Python libraries are commonly used for web scraping tasks, including Instagram data extraction. Key libraries include requests for handling HTTP requests, Beautiful Soup for parsing HTML/XML content, and Selenium for interacting with dynamic websites. Choosing the right library depends on the complexity of the data and the website’s structure.
Installation and Import
Before using these libraries, you need to install them. This is easily accomplished using pip, Python’s package installer. The following commands demonstrate how to install the necessary packages.
Learning how to download Instagram data with Python can be super helpful, especially if you’re into data analysis. But did you know that you can also save money on online purchases by using coupon and cashback platforms? Coupon and cashback platforms can really boost your savings, and Python can be used to find those deals too.
It’s a great way to automate your savings research and make sure you’re getting the best possible deals. So, if you’re looking to use Python to download Instagram data, the methods and processes are similar to other data retrieval methods.
pip install requests beautifulsoup4 selenium
After installation, you can import these libraries into your Python script:
import requests from bs4 import BeautifulSoup from selenium import webdriver
Library Strengths and Weaknesses
Each library has unique strengths and weaknesses when it comes to Instagram data extraction. Understanding these characteristics will help you choose the most suitable library for your specific needs.
Library | Strengths | Weaknesses |
---|---|---|
requests | Handles HTTP requests efficiently, suitable for static websites. Simple and easy to use for basic tasks. | Ineffective for dynamic websites (like Instagram), requires additional tools for complex tasks. Does not handle JavaScript rendering. |
Beautiful Soup | Excellent for parsing HTML/XML content. Extracts structured data from the parsed HTML. | Not suitable for interacting with dynamic web pages. Needs other tools for handling JavaScript. Does not handle browser interactions. |
Selenium | Handles dynamic websites, interacts with JavaScript, and emulates browser behavior. Useful for sites that require JavaScript rendering for data to be visible. | Slower than other libraries. More complex to set up and use. Requires a web driver for specific browsers. |
Comparison of Usage
The choice of library significantly impacts how you approach Instagram data extraction. Using requests
is straightforward for basic data, but limited for dynamic content. Beautiful Soup
excels at extracting data from the HTML structure after requests
retrieves the page. Selenium
is ideal for extracting data from dynamic sites like Instagram, which require JavaScript rendering.
The trade-offs between simplicity, speed, and functionality are important to consider.
Accessing Instagram API
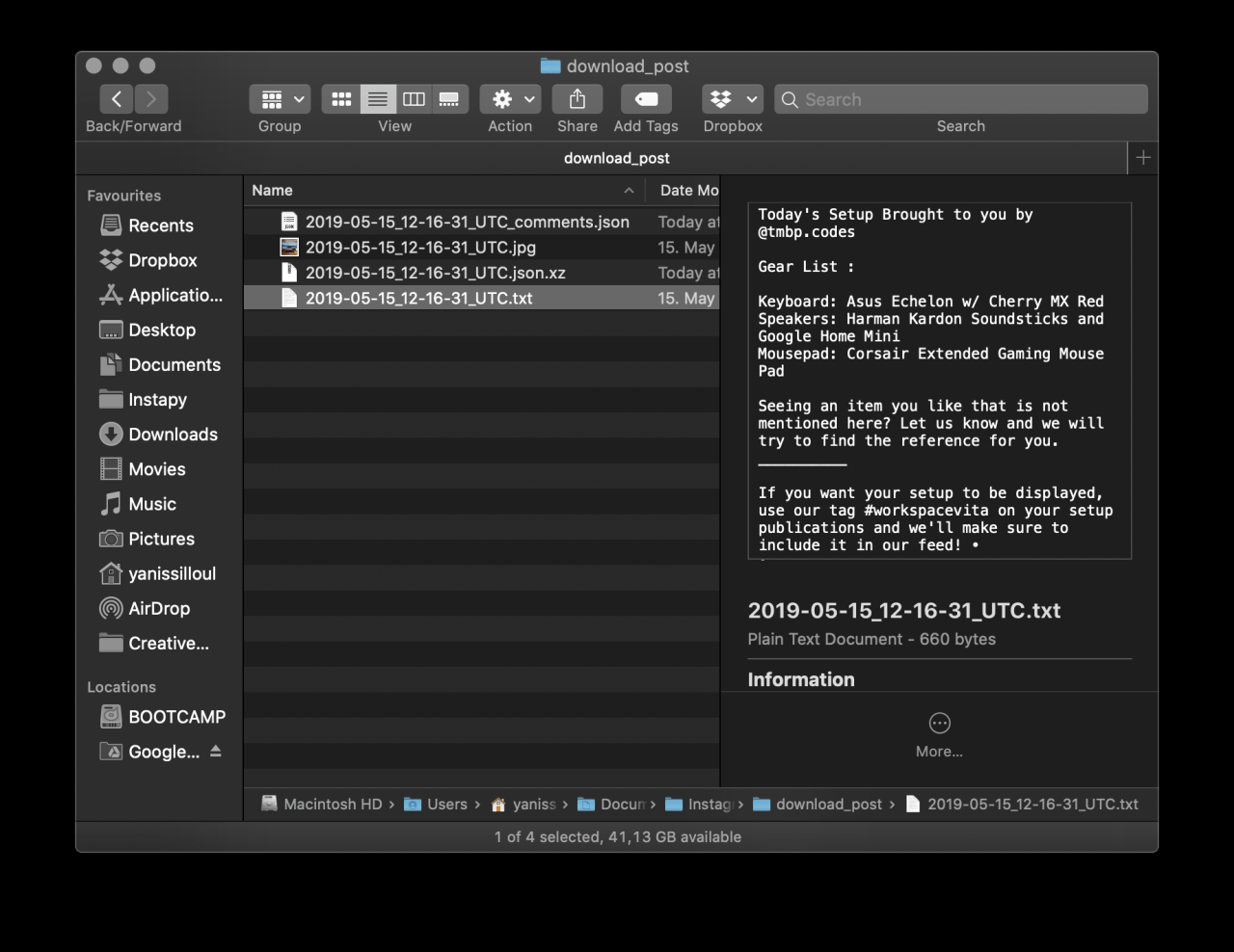
Instagram’s API is the gateway to accessing its data programmatically. This allows developers to gather information about users, posts, stories, and more, without relying on scraping. Understanding the API and its authentication process is crucial for ethical and effective data extraction.The Instagram API provides structured data in a format that’s easily parsed and processed by Python scripts. This structured approach, in contrast to scraping, ensures data integrity and stability, crucial for reliable analysis.
Ever wanted to snag all your Instagram photos and videos? Learning how to download Instagram data using Python is a fantastic way to do just that. But while you’re at it, why not explore self hosted photo backup alternatives? Self hosted photo backup alternatives give you complete control over your precious digital memories, ensuring they’re safely stored, and not just on someone else’s servers.
Once you’ve got your backup strategy sorted, you can confidently dive back into the world of Python-powered Instagram data retrieval.
Instagram API Authentication, How to download instagram data using python
Authenticating with the Instagram API is essential for authorized access to data. Instagram utilizes OAuth 2.0 for secure authentication, which involves obtaining an access token. This token grants your application permission to access specific data on behalf of the user.The authentication process typically involves redirecting the user to an Instagram authorization page, where they grant permission for your application to access their data.
Once authorized, the Instagram API returns an access token, which your Python script uses to make subsequent API requests.
Handling API Rate Limits and Pagination
Instagram API requests are subject to rate limits to prevent abuse and maintain system stability. Exceeding these limits can result in temporary or permanent blocks. Properly handling rate limits is crucial for maintaining access to the API.Implementing pagination is essential for retrieving large datasets, as Instagram APIs often return a limited number of results per request. Pagination allows you to fetch data in batches, ensuring you don’t miss any data points.
This approach is critical for handling potentially massive amounts of data and prevents API errors due to exceeding the data retrieval limits.
API Endpoints for Data Extraction
Various API endpoints are available for extracting different types of Instagram data. This table Artikels some key endpoints:
Data Type | Endpoint | Description |
---|---|---|
User Profile | /users/user-id | Retrieves information about a specific user, including their username, profile picture, bio, and other details. |
User Posts | /users/user-id/media | Fetches a user’s posts, including images, videos, captions, and timestamps. |
Post Comments | /media/media-id/comments | Retrieves comments associated with a specific post. |
User Stories | /users/user-id/stories | Retrieves information about a user’s stories, including the media contained within. |
This table provides a starting point; Instagram’s API documentation contains a comprehensive list of available endpoints for different data types.
Error Handling and Exception Management
Handling potential errors and exceptions during API interactions is critical for robust Python scripts. Instagram API responses often include error codes and messages, which can be used to troubleshoot issues and handle exceptions appropriately.Using `try-except` blocks is essential. This allows your script to gracefully manage situations like invalid access tokens, rate limit violations, or network connectivity problems, preventing script crashes and ensuring data integrity.
For instance, if a request times out, your script can retry the request or log the error.
Ever wanted to download your Instagram data? Python’s a fantastic tool for that! You can easily script a program to pull your photos, posts, and more. While we’re on the subject of data, did you know that Trump’s reciprocal tariffs had significant impacts on various goods? Understanding the fluctuating prices, like trump reciprocal tariffs prices , gives a clearer picture of the economic landscape.
Regardless, Python’s a powerful way to get your Instagram data organized and accessible.
Extracting Specific Data Types
Now that we’ve covered the groundwork of Instagram data extraction using Python, let’s dive into the nitty-gritty of retrieving specific data types. This section will detail how to extract user profiles, posts, comments, likes, and even Instagram Stories, providing practical examples and code snippets for each.
Extracting User Profiles
Fetching user information is a fundamental step in Instagram data extraction. This includes details like the username, bio, number of followers, and other relevant profile attributes. Accurate data retrieval allows for analysis of user engagement and audience demographics.
- To extract a user’s profile, you need their username. The Python code will typically involve making an API call to retrieve the user’s profile information. This often involves handling potential errors, such as incorrect usernames or API rate limits.
- Example: Retrieving the profile of a user named “elonmusk” would involve a specific API call, handling potential issues like invalid usernames and error messages.
Extracting Post Details
Extracting post details is crucial for understanding content shared on Instagram. This includes the post’s caption, image or video URLs, and other associated metadata. The process typically involves navigating the Instagram API and parsing the JSON responses.
- To extract a post’s details, you need the post’s ID. The Python code will typically involve making an API call to retrieve the post’s information. This data often needs careful parsing to extract the caption, image URL, and video URL (if applicable).
- Example: A post with ID “123456789” will return data like the caption text and the URL of the image or video.
Extracting Comments and Likes
Comments and likes provide insight into audience engagement with a specific post. Extracting this data requires identifying the post and then retrieving the related comments and like data from the API. Again, error handling is vital.
- To extract comments and likes, you need the post’s ID. The Python code will make an API call to retrieve the list of comments and the list of users who liked the post.
- Example: A post with ID “987654321” will return a list of comments and a list of users who liked the post.
Extracting Instagram Stories
Instagram Stories are a dynamic part of the platform, and extracting data from them requires a different approach than posts. The data structure for Stories often differs significantly from standard posts. Error handling is particularly important due to the ephemeral nature of stories.
- Extracting Stories involves accessing the relevant API endpoints and parsing the responses to extract the story content. This may include the images, videos, and timestamps associated with the story.
- Example: Extracting a story from a specific user requires accessing the user’s story feed and handling potential issues like story deletion or privacy settings.
Data Extraction Code Snippets (Illustrative)
Data Type | Python Code Snippet (Illustrative) | Error Handling (Illustrative) |
---|---|---|
User Profile | user_data = instagram_api.get_user_profile(username='elonmusk') |
try: ... except instagram_api.InstagramAPIError as e: print(f"Error: e") |
Post Details | post_data = instagram_api.get_post_details(post_id='123456789') |
try: ... except instagram_api.InstagramAPIError as e: print(f"Error: e") |
Comments & Likes | comments = instagram_api.get_post_comments(post_id='987654321') likes = instagram_api.get_post_likes(post_id='987654321') |
try: ... except instagram_api.InstagramAPIError as e: print(f"Error: e") |
Note: This table provides illustrative code snippets. Actual code may vary depending on the specific Instagram API library used.
Handling Data Storage and Formatting
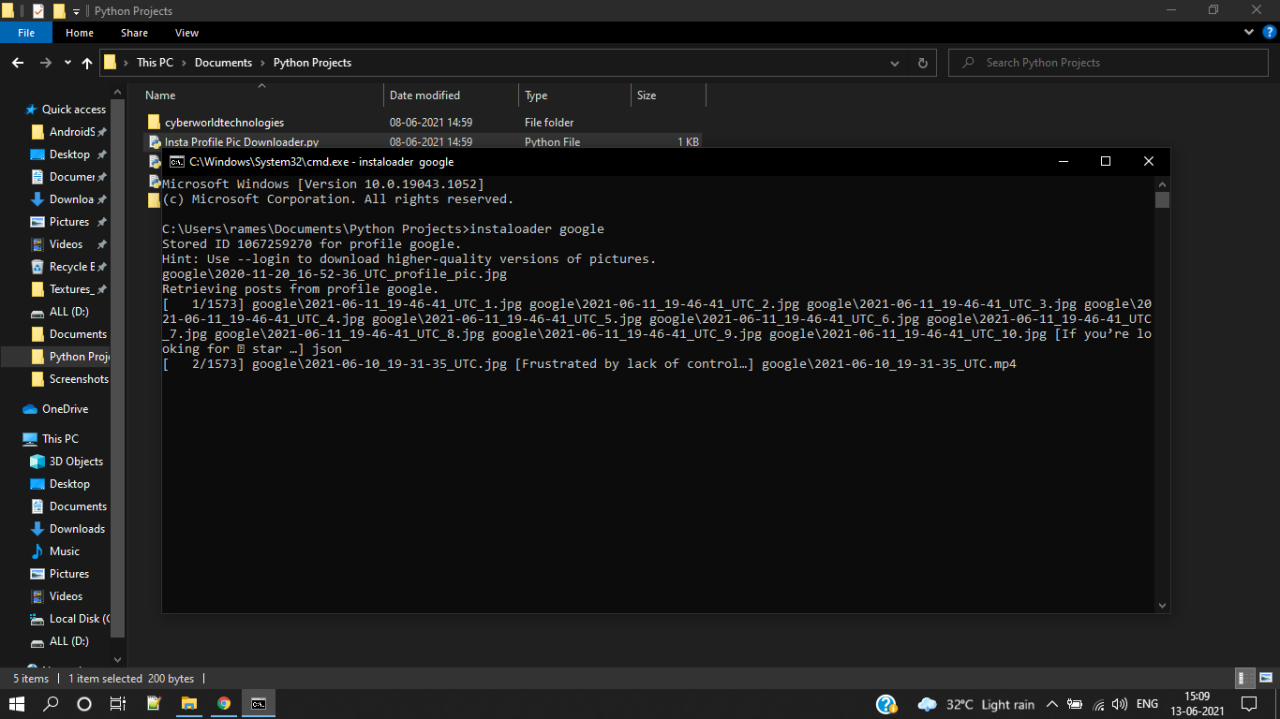
Storing and formatting your extracted Instagram data is crucial for analysis and future use. Properly structuring the data allows for easier manipulation and avoids confusion when revisiting the information later. This section details the various storage options and the essential steps to format the data for effective analysis.
Data Storage Options
Different storage formats cater to various needs. Choosing the right one depends on the volume of data, desired analysis, and your familiarity with the tools.
- CSV (Comma Separated Values): A simple text-based format, CSV is ideal for smaller datasets and straightforward analysis. It’s easily readable by spreadsheets and many programming languages. However, for complex datasets, CSV can become unwieldy and harder to manage.
- JSON (JavaScript Object Notation): A structured format, JSON is well-suited for larger datasets and more complex relationships between data points. It’s easily parsed and manipulated by various programming languages. Its hierarchical structure allows for nested data, making it excellent for representing interconnected data, such as comments with user information.
- Databases (e.g., PostgreSQL, MySQL): For very large datasets or projects requiring complex queries, databases provide a robust and efficient way to store and manage data. Databases offer features like indexing and efficient retrieval, which are invaluable for complex analyses.
Data Formatting and Cleaning
Formatting and cleaning the extracted data is essential for analysis. This involves standardizing formats, handling missing values, and ensuring data integrity.
- Standardizing Formats: Ensure consistent formats for dates, times, and other relevant fields. For example, if dates are extracted in various formats (e.g., MM/DD/YYYY, DD/MM/YYYY), convert them to a single format (e.g., YYYY-MM-DD) for consistency.
- Handling Missing Values: Missing data points can skew results. Decide how to handle these missing values (e.g., impute with the mean, median, or mode, or flag as missing). This is critical for reliable analysis.
- Data Validation: Validate the extracted data to identify and correct errors or inconsistencies. This could involve checking for data types, ranges, or logical relationships.
Handling Large Datasets
Extracting large volumes of Instagram data requires careful consideration.
- Chunking: Process the data in smaller chunks to avoid memory issues. This approach is especially useful for datasets that don’t fit into memory at once. Python libraries often provide tools for working with data in chunks.
- Parallel Processing: Distribute the processing of chunks across multiple processors or threads to significantly speed up the extraction and formatting process. This is often necessary when dealing with substantial volumes of data. Libraries like `multiprocessing` in Python are helpful for this.
Organizing Extracted Data
Organizing the data into a structured format is essential for efficient use. A structured format makes data easier to analyze, visualize, and report on.
- Creating a Schema: Define a schema or data model to structure the data. This schema Artikels the different fields and their data types. For example, a schema might include fields like ‘username’, ‘post_date’, ‘likes’, and ‘comments’.
- Creating Tables (for Databases): When using databases, create tables that reflect the schema. This allows for efficient data retrieval and querying. Databases provide a structured way to organize and manage the data.
Saving Data in a Specific Format
Saving the data in the chosen format is the final step.
- CSV Export: Libraries like `csv` in Python can be used to save extracted data to CSV files. The `to_csv()` method in pandas is a common approach for exporting dataframes to CSV format.
- JSON Export: Python’s `json` library allows you to save extracted data in JSON format. Libraries like `pandas` also have methods for exporting dataframes to JSON format.
- Database Insertion: Python libraries provide tools to insert data into databases. For instance, the `psycopg2` library is often used to interact with PostgreSQL databases.
Advanced Techniques and Considerations: How To Download Instagram Data Using Python
Instagram’s dynamic nature and robust anti-scraping measures demand advanced techniques for effective data extraction. This section explores strategies for navigating these challenges, ensuring ethical practices, and maintaining consistent data collection. Understanding these methods is crucial for building robust and reliable Python scripts for Instagram data extraction.Successfully extracting data from Instagram requires more than just basic API calls. Dynamic content, evolving website structures, and Instagram’s anti-scraping mechanisms necessitate careful planning and implementation of sophisticated techniques.
This includes understanding how to handle changing data formats, utilizing proxies effectively, and employing ethical data collection practices.
Dealing with Dynamic Content
Instagram frequently updates its website structure and uses JavaScript to dynamically load content. Standard HTTP requests often miss this crucial information. Employing libraries like Selenium, which can simulate a web browser, is necessary for capturing dynamically loaded data. This involves rendering JavaScript, allowing the Python script to access the full content of the page. Careful use of selectors, coupled with thorough understanding of the page’s structure, is vital.
Overcoming Anti-Scraping Measures
Instagram actively blocks scraping attempts. These measures include rate limiting, IP blocking, and complex anti-bot detection mechanisms. Implementing delays between requests, rotating IP addresses, and using user-agent spoofing are crucial for circumventing these measures. Utilizing a rotating proxy pool can help maintain a constant flow of data while avoiding immediate detection.
Using Proxies for Enhanced Scraping
Proxies act as intermediaries between the scraper and Instagram. Rotating proxies from reputable providers are essential to maintain a constant stream of data and avoid IP blocks. This ensures scraping efficiency and bypasses Instagram’s rate limits. Efficiently managing a proxy pool is essential for avoiding detection. This includes ensuring that the proxies are active and working correctly.
Handling Website Structure Changes
Instagram’s website is constantly evolving. To ensure the scraping script remains functional, implement robust methods to detect and adapt to changes. Use tools and libraries that allow for dynamic analysis of the page structure, and adapt the script’s selectors accordingly. Testing and validation are key to adapting to evolving structures and maintaining data accuracy.
Ethical and Legal Data Collection Practices
Ethical data collection is paramount. Respect Instagram’s terms of service, avoid overwhelming their servers with requests, and ensure the data is used responsibly. Explicitly stating the purpose of the extraction and the terms of use of the data are important. Ensure the data is used in accordance with all relevant regulations and laws. This includes adhering to the principles of privacy and data security.
Always obtain explicit consent when collecting personal information.
Example Implementation
Let’s dive into a practical example of extracting Instagram data using Python. This comprehensive example will guide you through the entire process, from setting up the environment to storing the extracted data. We’ll use the `instagrapi` library, which is a robust and well-documented option for interacting with the Instagram API.
Setting Up the Environment
Before running the code, ensure you have the necessary Python libraries installed. The `instagrapi` library is essential for interacting with the Instagram API. Install it using pip:
pip install instagrapi
Also, install other necessary libraries if needed (e.g., for data manipulation or storage).
Code Implementation
The following code snippet demonstrates the complete process of downloading Instagram data for a specific user. Remember to replace the placeholder values with your actual username and password.
“`pythonimport instagrapi# Replace with your username and passwordusername = “your_username”password = “your_password”# Initialize the Instagram API clientclient = instagrapi.Client()# Log in to Instagramclient.login(username, password)# Specify the Instagram usertarget_user = username# Fetch the user’s profileuser_profile = client.user_info(username=target_user)# Extract user’s postsposts = client.user_medias(user_profile.pk)# Process the posts (e.g., extract captions, dates, likes, etc.)for post in posts: print(f”Post Caption: post.caption”) print(f”Post Date: post.taken_at”) print(f”Post Likes: post.likes”) # …
add other data extraction here …# Store the extracted data (e.g., to a CSV file or database)# Example:# with open(‘instagram_data.csv’, ‘w’, encoding=’utf-8′) as csvfile:# # … write the extracted data to the CSV file …“`
Step-by-Step Implementation
The following table Artikels the crucial steps involved in the data extraction process, along with explanations.
Step | Description |
---|---|
1. Import Libraries | Import the necessary Python libraries, including `instagrapi`. |
2. Login | Use the `client.login()` method to authenticate with your Instagram account. |
3. Target User | Specify the Instagram user whose data you want to extract. |
4. Fetch Profile | Retrieve the user’s profile information using `client.user_info()`. |
5. Extract Posts | Retrieve the user’s posts using `client.user_medias()`. |
6. Data Processing | Extract specific data points from each post, such as captions, likes, and dates. |
7. Data Storage | Store the extracted data in a structured format, such as a CSV file or a database. |
Adapting the Example
This example can be adapted for various use cases. For instance, you could:
- Extract data for multiple users by iterating through a list of usernames.
- Filter posts based on specific criteria (e.g., date range, hashtags).
- Store the extracted data in a different format (e.g., JSON, XML).
- Use a more advanced data storage method (e.g., a relational database).
Final Wrap-Up
In conclusion, extracting Instagram data using Python offers a wealth of possibilities for analysis and insights. This guide has equipped you with the knowledge and tools to navigate the process, from understanding the necessary libraries to managing data storage and handling complex scenarios. Remember to always respect Instagram’s terms of service and adhere to ethical data collection practices. Now go forth and unlock the power of your Instagram data!