Can We Trust AI in Emerging Tech?
Can we trust AI navigating emerging technology? This question is crucial as AI increasingly shapes our world. From self-driving cars to personalized medicine, AI is rapidly integrating into various sectors. This exploration delves into the complexities of trust, examining AI’s capabilities, potential pitfalls, and strategies for building confidence in its use across emerging technologies. We’ll examine the factors influencing trust, the risks associated with specific applications, and what developers and policymakers can do to foster a future where AI is both powerful and reliable.
Defining Trust in AI Navigation: Can We Trust Ai Navigating Emerging Technology
Trust in AI, particularly when it navigates emerging technologies, is a multifaceted concept that goes beyond simple reliability. It encompasses a range of user expectations and perceptions about the AI’s behavior, capabilities, and the system’s overall impact. This trust is crucial for widespread adoption and acceptance of AI-driven systems. The increasing reliance on AI in various sectors necessitates a thorough understanding of the factors that contribute to this trust.Defining trust in AI navigation requires considering the specific context and the user’s expectations.
Trust is not a binary state; it’s a spectrum, ranging from complete skepticism to unwavering confidence. This spectrum is influenced by various factors, including the perceived reliability, transparency, and accountability of the AI system. Understanding these facets allows for the design and implementation of AI systems that inspire user trust and foster a positive user experience.
Different Facets of Trust
Trust in AI systems, like trust in human experts, rests on several key pillars. Reliability, the consistency and accuracy of the AI’s performance, is paramount. Transparency, the clarity and explainability of the AI’s decision-making processes, builds user confidence. Accountability, the ability to identify and address errors or biases within the AI system, is essential for maintaining trust over time.
These three elements work together to create a robust framework for building user trust in AI-driven navigation systems.
Relationship Between Trust and User Acceptance
A high level of trust is directly correlated with the acceptance of AI-driven systems. Users are more likely to adopt and utilize AI systems they perceive as trustworthy. Conversely, low levels of trust can lead to resistance and skepticism, hindering the widespread adoption of potentially beneficial technologies. For example, autonomous vehicles require a high degree of trust from drivers and the public for widespread deployment.
User acceptance is dependent on the public’s confidence in the vehicle’s ability to navigate safely and predictably.
Trust in AI vs. Trust in Human Experts, Can we trust ai navigating emerging technology
While trust in human experts often relies on established reputations and personal interactions, trust in AI requires a different approach. Trust in human experts can be based on experience and demonstrable competence. Trust in AI, however, needs to be built on demonstrable reliability, transparent processes, and accountability mechanisms. For instance, a medical doctor might earn trust through years of experience and positive patient outcomes.
An AI medical diagnosis system, however, needs to prove its reliability and transparency through rigorous testing and verifiable results. The factors for trust in AI and human experts differ significantly, reflecting the unique nature of each.
Types of Trust and Implications for AI Navigation
Type of Trust | Definition | Implications for AI Navigation |
---|---|---|
Reliability Trust | Confidence in the AI’s consistent and accurate performance. | AI systems must demonstrate high accuracy and stability in their navigation tasks. This includes minimizing errors and unexpected behaviors. |
Transparency Trust | Understanding how the AI system makes decisions. | AI systems should provide clear explanations for their navigation choices. This could involve visualizing the decision-making process or providing detailed reports. |
Accountability Trust | The ability to identify and address issues within the AI system. | Mechanisms for identifying and rectifying errors or biases in the AI’s navigation algorithms are crucial. This includes clear lines of responsibility and effective redressal procedures. |
Competence Trust | Belief in the AI’s capabilities to handle complex situations. | AI systems need to be tested rigorously in diverse and challenging navigation scenarios. This involves demonstrating proficiency in various environments and handling unexpected situations. |
Assessing AI’s Capabilities in Emerging Technologies
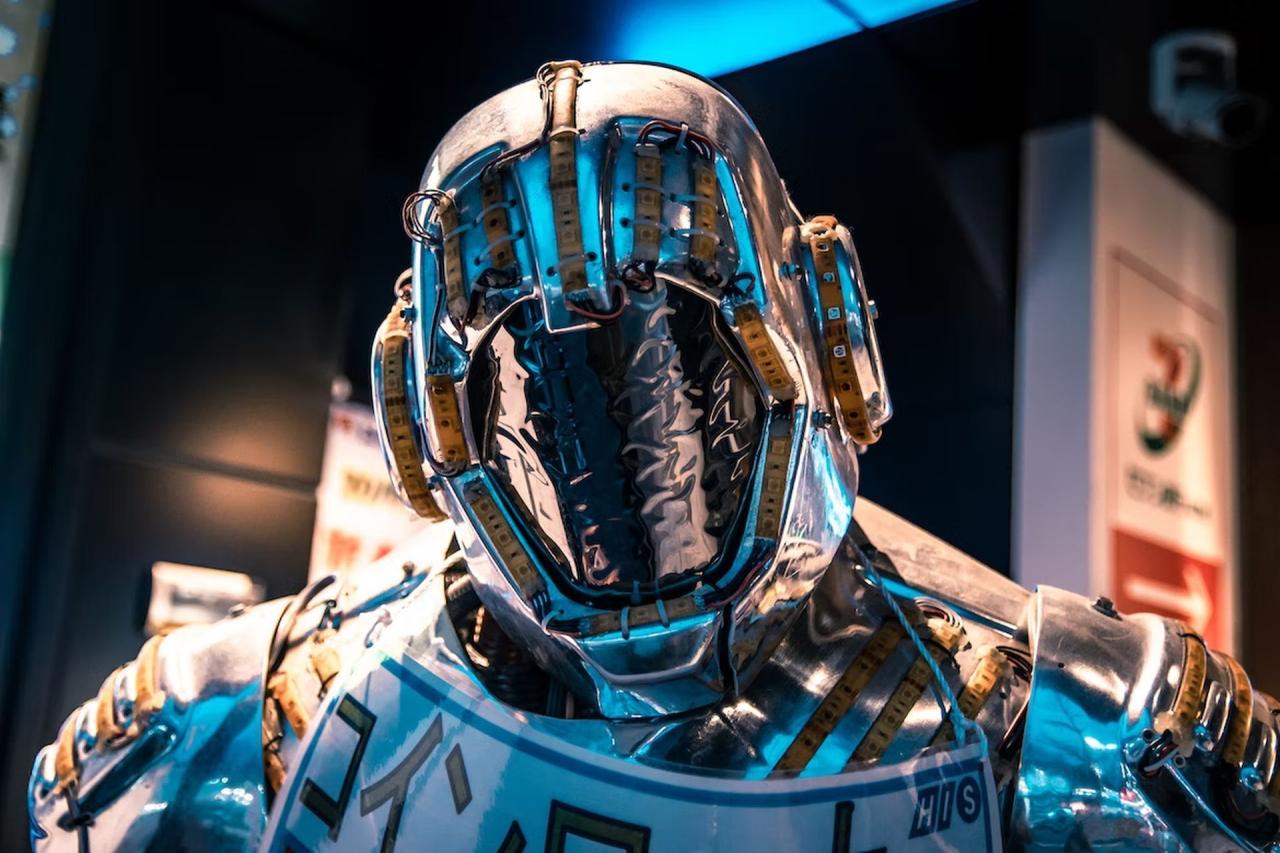
AI navigation is rapidly transforming various sectors, from autonomous vehicles to healthcare. Understanding the capabilities, limitations, and potential risks of AI in these emerging technologies is crucial for responsible development and deployment. This exploration delves into the specific applications of AI, highlighting successful and unsuccessful implementations, and ultimately aims to provide a nuanced perspective on its role in shaping the future.AI is now integral to a wide array of emerging technologies.
Honestly, trusting AI to navigate the complexities of emerging technology feels a bit like trusting a rookie Steph Curry to lead the Golden State Warriors to a championship—we’ve got some promising signs, but the ultimate success is still uncertain. While Steph Curry’s impact on the court is undeniable, and his book on the Warriors’ journey is sure to be an interesting read, Steph Curry Warriors book NBA might not offer all the answers we need to predict AI’s future success in these rapidly evolving fields.
Ultimately, the question of AI reliability in emerging tech remains a fascinating, and frankly, a little bit unpredictable one.
Its capabilities extend beyond simple tasks, enabling complex decision-making in dynamic environments. However, the application of AI is not without its challenges. Understanding the limitations and potential risks associated with each technology is essential for responsible implementation and mitigating potential harm.
Autonomous Vehicles
AI plays a pivotal role in autonomous vehicles, enabling them to perceive and react to their environment. Sophisticated algorithms process sensor data from cameras, radar, and lidar to create a comprehensive understanding of the surroundings. This allows vehicles to navigate roads, avoid obstacles, and make decisions in real-time.The specific capabilities of AI in autonomous vehicles include object detection, traffic signal recognition, lane keeping, and dynamic route planning.
These capabilities are being continuously refined through advancements in machine learning algorithms.Limitations include the robustness of AI in handling unforeseen situations, such as extreme weather conditions or unexpected pedestrian behavior. The reliance on data for training can lead to biases and difficulties in adapting to unfamiliar environments. Safety concerns are paramount, and the potential for accidents remains a significant risk.Successful implementations of AI in autonomous vehicles are evident in pilot programs and limited-scale deployments, demonstrating the potential for improved safety and efficiency.
However, challenges remain in terms of public acceptance, regulatory frameworks, and the development of robust safety systems. Examples of unsuccessful implementations include incidents where AI systems failed to anticipate or react appropriately to unexpected events.
Healthcare
AI is revolutionizing healthcare by enabling more accurate diagnoses, personalized treatment plans, and improved patient outcomes. AI-powered diagnostic tools can analyze medical images, such as X-rays and CT scans, to identify anomalies with higher accuracy and speed than traditional methods.Specific AI capabilities in healthcare include image analysis, drug discovery, personalized medicine, and predictive modeling for disease outbreaks. Machine learning algorithms are used to identify patterns and anomalies in medical data, leading to more precise diagnoses and treatment recommendations.Limitations include the need for large, high-quality datasets for training AI models, potential biases in these datasets, and concerns about patient privacy and data security.
The ethical implications of using AI in healthcare also require careful consideration.Examples of successful implementations include the use of AI in cancer detection, where algorithms have shown promise in identifying early-stage tumors with greater accuracy. Unsuccessful implementations include instances where AI systems failed to adequately account for variations in patient demographics or specific medical conditions, leading to inaccurate diagnoses or treatment recommendations.
Finance
AI is transforming the financial sector by automating tasks, detecting fraud, and improving risk management. AI-powered systems can analyze vast amounts of financial data to identify patterns and anomalies that might indicate fraudulent activity.Specific capabilities include fraud detection, algorithmic trading, risk assessment, and customer service automation. AI can quickly process and analyze data, leading to more efficient and effective decision-making in the financial sector.Limitations include the risk of bias in AI algorithms, the potential for algorithmic trading to exacerbate market volatility, and the need for robust regulatory frameworks to ensure responsible use.
The ethical implications of using AI in finance, particularly in relation to algorithmic trading, are significant and require ongoing debate.Successful implementations of AI in finance include the use of AI for fraud detection, where algorithms have proven effective in identifying and preventing fraudulent transactions. Unsuccessful implementations involve instances where AI models failed to adapt to changing market conditions or were susceptible to manipulation.
Table Comparing AI Capabilities Across Technologies
Technology | AI Capabilities | Limitations | Risks | Examples of Success | Examples of Failure |
---|---|---|---|---|---|
Autonomous Vehicles | Object detection, navigation, route planning | Unforeseen situations, bias in training data | Accidents, safety concerns | Pilot programs, limited deployments | Incidents of AI systems failing to react appropriately |
Healthcare | Image analysis, drug discovery, personalized medicine | Data quality, biases, privacy | Inaccurate diagnoses, treatment errors | Cancer detection, personalized treatment | Inadequate consideration of patient demographics |
Finance | Fraud detection, algorithmic trading, risk assessment | Bias, market volatility, regulatory issues | Fraudulent activities, market manipulation | Fraud detection, algorithmic trading | AI models failing to adapt to changing market conditions |
Understanding the Challenges to Trust
Navigating the emerging landscape of AI technology requires a nuanced understanding of the factors that influence user trust. Trust in AI systems, particularly for critical applications like navigation, hinges on several key elements. This includes the fairness, transparency, and reliability of the algorithms themselves, along with the potential for errors and failures in the system. Understanding these challenges is crucial for developing and deploying AI navigation systems that are both effective and trustworthy.
Bias, Fairness, and Ethical Concerns
AI systems learn from data, and if that data reflects existing societal biases, the AI system will inevitably perpetuate and potentially amplify those biases. For instance, if a navigation system is trained on data predominantly from one demographic group, it might not accurately reflect the conditions and needs of other groups, leading to unfair or inaccurate routes for them.
This inherent bias can have serious implications, affecting safety and access to resources for different user groups. Ensuring fairness and ethical considerations are central to building trustworthy AI navigation systems, demanding careful data selection and algorithm design processes. Ethical guidelines and robust testing procedures are necessary to mitigate potential biases and ensure equitable outcomes.
Algorithmic Opacity and Lack of Explainability
Many AI systems, especially those using deep learning, operate as “black boxes.” This opacity, or lack of explainability, makes it difficult for users to understand how the system arrives at a particular navigation recommendation. Without understanding the reasoning behind a decision, users may struggle to trust the system, especially when faced with unexpected or seemingly illogical routes. This lack of transparency can erode trust, as users feel a lack of control and accountability in the system.
Honestly, trusting AI to navigate the complexities of emerging technology is a bit of a gamble. It’s like setting up a new operating system, like trying to run Windows 11 in a virtual box, windows 11 in virtual box. You’re hoping everything works smoothly, but there are always potential glitches and unknowns. So, while AI holds incredible promise, we need to approach its role in shaping the future with a healthy dose of caution and careful consideration.
Furthermore, this opacity can hinder the identification and resolution of errors, potentially leading to dangerous situations.
Impact of Potential Failures or Errors
AI navigation systems, like any technology, are susceptible to errors. These errors can manifest as incorrect route suggestions, missed destinations, or even complete system failures. The implications of these errors can range from inconvenience to serious safety concerns. For example, a navigation system failing during a critical situation, like a natural disaster or emergency response, could have catastrophic consequences.
Users need to have confidence that the AI system will perform reliably and accurately, especially in high-stakes situations. Robust testing, validation, and error-handling mechanisms are vital to minimizing the impact of failures.
Potential Issues Eroding User Trust
- Inaccurate or unreliable information: AI systems relying on outdated or inaccurate data sources will provide flawed recommendations. This can lead to users getting lost, missing important landmarks, or wasting time on ineffective routes.
- Unforeseen or unpredictable behavior: An AI system that behaves erratically or in a way that users cannot anticipate will erode trust. Sudden changes in route suggestions without apparent reason, or the system choosing seemingly illogical paths, will raise concerns about reliability and safety.
- Lack of user control or customization: If users have limited ability to customize or influence the navigation process, they may feel powerless and distrust the system’s decisions.
- Privacy concerns: Navigation systems often collect user data. If users perceive that their data is not handled securely or ethically, it can lead to a decline in trust.
- Inadequate communication and support: Clear communication and support are crucial to addressing user concerns and issues with the system. Lack of support or difficulty in understanding error messages can negatively impact user trust.
Strategies for Building Trust in AI
Building trust in AI is crucial for its widespread adoption and responsible integration into society. The ability to navigate emerging technologies relies heavily on the public’s confidence in the systems. Without trust, concerns and resistance will hinder progress. This necessitates a multifaceted approach that prioritizes transparency, accountability, and public understanding. Strategies for building trust are not simply about appeasing the public; they are about ensuring that AI systems are reliable, fair, and beneficial to all.
Enhancing Transparency and Explainability in AI Systems
Transparency in AI systems is essential for building trust. Users need to understand how the system arrives at its decisions. This involves making the inner workings of the algorithms accessible and understandable. Black box approaches, where the decision-making process is opaque, erode trust. Explainable AI (XAI) techniques provide crucial insights into the reasoning behind AI predictions, enabling scrutiny and verification.
Furthermore, clear documentation of data sources and model parameters further strengthens transparency.
Promoting Accountability and Responsibility in AI Development
Accountability in AI development is paramount. Clear lines of responsibility for errors and biases are vital. Establishing frameworks for auditing AI systems and defining standards for ethical development are essential. Furthermore, incorporating human oversight and intervention where appropriate can mitigate potential risks. This proactive approach ensures that AI systems are not simply tools, but are developed and deployed responsibly.
Fostering Public Awareness and Understanding of AI’s Capabilities and Limitations
Public awareness and understanding are critical to building trust. Educating the public about AI’s capabilities and limitations is crucial. This includes highlighting both the potential benefits and potential risks of AI. Open dialogue and accessible information can help dispel myths and fears surrounding AI. Educational programs, workshops, and public forums can play a pivotal role in achieving this goal.
It’s also important to address misconceptions about AI’s capabilities and its potential impact on jobs, society, and the economy.
Addressing Potential Biases and Ensuring Fairness in AI
AI systems trained on biased data can perpetuate and amplify existing societal biases. Addressing these biases is crucial to ensuring fairness. Careful data selection and pre-processing are essential steps in mitigating bias. Regular audits and ongoing monitoring can help identify and correct biases that emerge during operation. Techniques like fairness-aware machine learning algorithms are being developed to address this challenge head-on.
It is important to consider diverse perspectives in the design and development process.
Specific Steps for Developers to Build Trust
Category | Specific Steps |
---|---|
Transparency |
|
Accountability |
|
Public Awareness |
|
Bias Mitigation |
|
Illustrative Examples of Trust in Action
Building trust in AI navigation systems for emerging technologies requires demonstrable reliability and transparency. Successful implementations showcase how trust is not a one-time achievement, but a continuous process requiring adaptation and improvement. These examples highlight the key strategies employed to foster trust, along with the challenges encountered and the lessons learned.
Figuring out if AI can be trusted with emerging tech is a serious question. It’s a bit like wondering if the best dayz hosting for gamers best dayz hosting for gamers will provide a smooth experience. Both hinge on a reliable foundation. Ultimately, trust in AI navigating these new frontiers depends on the ethical frameworks and responsible development that underpins its use.
Autonomous Vehicle Navigation Systems
Autonomous vehicles represent a prime example of emerging technology requiring robust AI navigation. Trust in these systems hinges on their ability to make safe and reliable decisions in complex environments. For instance, systems using deep learning for object detection and lane keeping have demonstrated a significant reduction in accidents compared to human-driven vehicles in controlled testing environments.
- Strategies for Building Trust: Rigorous testing protocols, including simulations of diverse scenarios, are crucial. Data transparency, where the algorithms’ decision-making processes are documented and accessible, builds trust. Open communication channels with the public about system limitations and ongoing development are also essential.
- Challenges Faced: Unexpected events, like pedestrians or animals crossing the road, can be difficult for AI to anticipate. Maintaining trust requires addressing these edge cases and continuously updating the system’s knowledge base.
- Lessons Learned: The development of robust safety mechanisms and comprehensive testing procedures are critical. Openness and transparency about the limitations and development process are paramount for fostering public trust.
AI-Powered Medical Diagnostics
AI is increasingly used in medical diagnostics, offering the potential to improve accuracy and speed. Trust in AI-driven diagnostic tools rests on their ability to provide reliable and unbiased results, potentially impacting patient outcomes.
- Strategies for Building Trust: Demonstrating high accuracy and reliability through extensive clinical trials and validation studies is paramount. Transparency about the algorithm’s training data and its limitations is essential. Clear communication about the role of the AI and the human physician in the diagnostic process fosters trust.
- Challenges Faced: Addressing potential biases in the training data and ensuring equitable access to AI-powered diagnostics are critical challenges. Maintaining the accuracy of these systems in the face of evolving medical knowledge and new conditions requires ongoing updates and refinement.
- Lessons Learned: Careful data selection and rigorous validation procedures are critical for minimizing biases. Establishing clear protocols for human oversight and intervention can mitigate the risk of errors.
Case Study: AI-Powered Crop Monitoring
Consider a case where an AI system monitors crop health using satellite imagery. The system identifies areas with potential stress, such as drought or nutrient deficiencies. Farmers use this data to adjust irrigation and fertilization strategies, potentially increasing yields.
- Trust Building: The system’s ability to accurately predict crop stress based on real-world data builds trust. Clear communication about the data used and the system’s limitations assures farmers. Demonstrating a consistent record of accurate predictions further strengthens trust.
- Trust Erosion: If the system consistently misidentifies stress factors or provides unreliable data, farmer trust is eroded. Lack of transparency regarding the algorithm’s decision-making process could lead to distrust and skepticism.
- Lessons Learned: The system’s ability to adapt to different conditions and provide actionable insights is critical. Regular feedback loops and ongoing refinement of the system based on farmer input are vital.
A Trustworthy AI Navigation System for Autonomous Farming Equipment
Imagine an AI system that controls autonomous tractors, monitoring soil conditions and adjusting planting and harvesting schedules. This system would utilize a combination of sensor data (soil moisture, nutrient levels), satellite imagery, and weather forecasts.
- Trustworthy Design: This system prioritizes data integrity and reliability, incorporating multiple data sources. The system’s actions are transparent and auditable. A clear protocol for human intervention in case of unexpected events is established.
- Key Components: Redundant sensor systems, rigorous calibration procedures, and a robust data validation pipeline are critical components. Clear communication channels for reporting issues and updating the system are essential.
Future Considerations for Trust in AI
The rapid advancement of AI, particularly in navigation, necessitates a careful examination of the evolving trust landscape. As AI systems become more sophisticated and integrated into various aspects of our lives, understanding the future of trust in these technologies is crucial. This involves anticipating potential pitfalls and proactively developing strategies to ensure reliability and transparency.AI navigation, from self-driving cars to autonomous drones, promises unprecedented efficiency and convenience.
However, the inherent complexity of these systems and their reliance on vast datasets raises critical questions about the very nature of trust. How can we ensure that these systems operate safely and ethically, and build public confidence in their capabilities? This section delves into the future challenges and considerations surrounding trust in AI navigation.
Evolving Nature of Trust in Rapidly Changing Technology
The pace of technological change is accelerating, making trust in AI a dynamic concept. Public trust in emerging technologies often evolves based on experiences and perceived risks. What was considered a futuristic marvel yesterday may become a commonplace technology tomorrow, and public acceptance may change with the changing landscape of adoption. Examples include early adoption of mobile phones and the subsequent integration of social media platforms.
These transformations highlight the need for continuous evaluation and adaptation of trust metrics and strategies to maintain public confidence in AI.
Potential Future Challenges to Trust in AI Navigation
Several factors could erode public trust in AI navigation. Unexpected malfunctions or accidents involving AI-powered vehicles or drones could significantly damage public perception. Moreover, the potential for bias in training data or algorithmic design could lead to unfair or discriminatory outcomes, further jeopardizing trust. The lack of transparency in AI decision-making processes also raises concerns about accountability and responsibility.
Furthermore, the reliance on AI for critical tasks may lead to a sense of vulnerability and dependence, which could, in turn, erode public trust if the system fails to meet expectations.
Role of Regulation and Policy in Shaping Trust in AI
Clear regulations and policies are essential for building trust in AI navigation. Well-defined guidelines on data privacy, algorithmic transparency, and safety standards are necessary to prevent misuse and ensure responsible development and deployment. These regulations must be adaptable to the rapidly evolving nature of AI technology to address emerging challenges. Regulatory frameworks should consider the specific context of AI navigation applications, recognizing that self-driving cars face different challenges than autonomous drones.
This proactive approach to regulation can help foster public confidence and minimize potential risks.
Scenarios where AI’s Navigation Could Profoundly Affect Trust in Emerging Technology
The integration of AI navigation into various sectors, including transportation, healthcare, and infrastructure, could significantly affect trust in emerging technologies. A critical scenario is a widespread deployment of self-driving cars that results in a higher accident rate compared to human-driven vehicles. This could severely damage public confidence in AI navigation systems and potentially halt further development and deployment.
Conversely, if AI navigation proves its safety and efficiency in diverse applications, it could positively impact trust in other emerging technologies, fostering broader adoption and acceptance. The interplay of factors such as accident rates, data security breaches, and the perceived fairness of AI algorithms will dictate the extent of trust in the future.
Potential Evolution of Trust Metrics for AI Systems
Trust metrics for AI systems need to evolve to reflect the complex and dynamic nature of trust. Traditional metrics, such as accuracy and reliability, are important but insufficient. Future metrics should also encompass factors like transparency, fairness, accountability, and resilience. These metrics should be continuously evaluated and adapted to address the evolving challenges and opportunities in the field.
Furthermore, the development of robust evaluation frameworks that include public input and diverse perspectives is critical for ensuring trust in AI navigation.
Final Conclusion
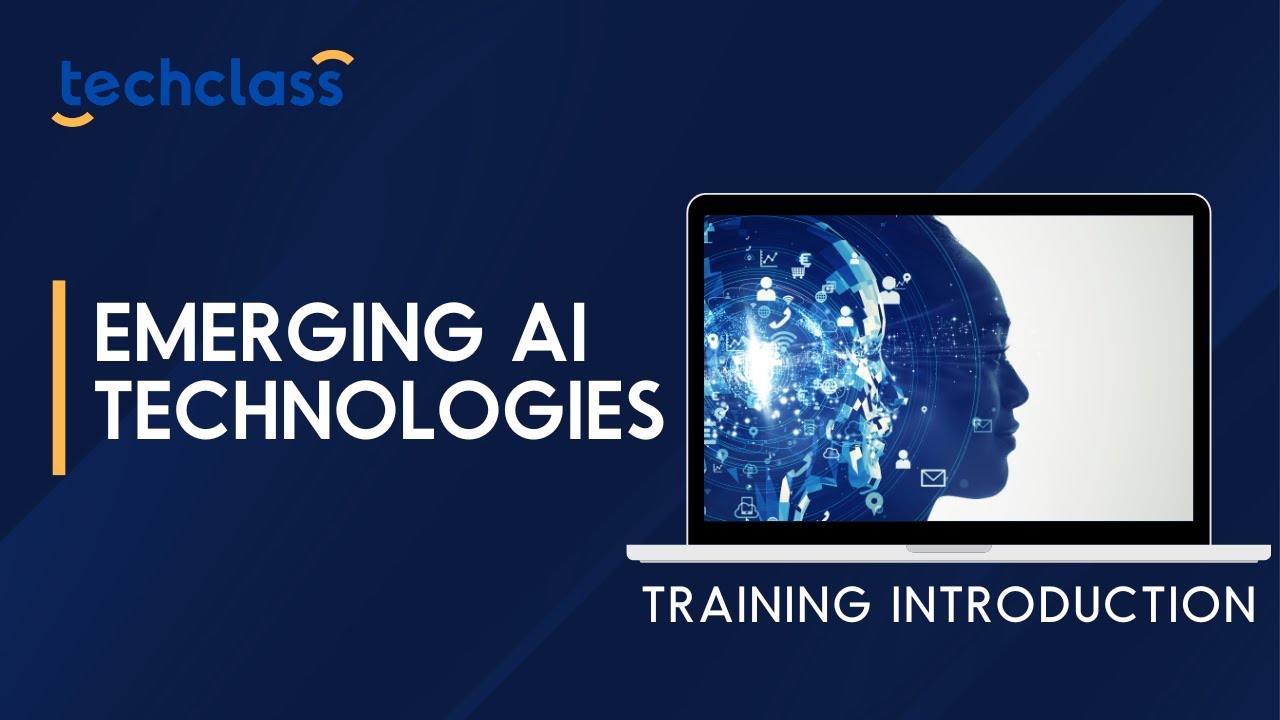
In conclusion, navigating the future of AI in emerging technology demands a nuanced approach to trust. While AI’s potential is undeniable, careful consideration of its limitations, biases, and potential for error is paramount. By focusing on transparency, accountability, and ethical development, we can pave the way for a future where AI benefits humanity while maintaining trust and safeguarding against potential harms.
This complex issue requires ongoing dialogue and a collaborative effort from developers, researchers, policymakers, and the public to ensure AI’s responsible integration into society.