Health Care AI Expensive Humans Needed
Health care AI requires expensive humans. This isn’t about robots replacing doctors, but understanding the substantial financial and human resources needed to successfully integrate AI into healthcare. From hefty implementation costs to ongoing maintenance, and the specialized expertise required to make it work, the reality is more complex than a simple technological upgrade.
The sheer cost of implementing AI systems in healthcare is substantial, ranging from initial setup to ongoing maintenance. Different AI applications, like diagnostic tools or treatment assistance, carry varying price tags. Beyond the financial investment, the human element is equally critical. Skilled personnel are needed for everything from developing and training the AI to ensuring its integration with existing systems, all while navigating complex ethical considerations.
The Cost of Implementation
Implementing AI in healthcare promises significant improvements, but the financial implications are substantial. Beyond the initial investment, ongoing maintenance, upgrades, and potential retraining of personnel require careful consideration. The costs vary widely depending on the specific AI application and the scale of the implementation. Understanding these factors is crucial for any organization contemplating integrating AI into its healthcare operations.The cost of implementing AI in healthcare is multifaceted, encompassing not only the purchase price of the software and hardware but also the necessary infrastructure, personnel training, data acquisition, and ongoing maintenance.
This comprehensive approach ensures the AI system operates effectively and provides value over time. Different pricing models reflect the varying needs and complexities of different healthcare settings.
Initial Investment Costs
The initial investment for AI healthcare projects can range from a few thousand dollars for simple applications to millions for large-scale, complex systems. Factors such as the sophistication of the AI algorithms, the size of the data sets used, and the required computing infrastructure significantly influence the cost. For example, a basic AI system for analyzing patient images might cost a few thousand dollars, while a sophisticated AI system for predicting disease outbreaks could cost millions.
Cloud-based solutions often have lower initial costs, but ongoing subscription fees should be factored in.
Maintenance and Upgrade Costs
Ongoing maintenance and upgrades are critical for maintaining the efficacy and performance of AI systems. Software updates, hardware replacements, and staff training to adapt to evolving AI technologies contribute to these costs. The frequency and complexity of updates depend on the specific AI system and the rate of technological advancements in the field. For instance, a system relying on deep learning algorithms might require more frequent updates compared to a system using simpler statistical models.
Upgrades may be necessary to accommodate increasing data volumes or to integrate with new healthcare platforms.
Pricing Models
Various pricing models for AI-powered healthcare solutions exist, including subscription-based models, pay-per-use models, and one-time purchase models. Subscription models provide access to the AI platform and ongoing maintenance, while pay-per-use models charge for specific functionalities or usage. A one-time purchase often applies to simpler, more standalone AI tools. Each model has its own advantages and disadvantages, and the optimal choice depends on the organization’s specific needs and financial resources.
Return on Investment (ROI)
The potential return on investment (ROI) for AI implementations in healthcare is significant. AI can automate tasks, improve diagnostic accuracy, and personalize treatment plans, leading to cost savings and improved patient outcomes. These improvements translate to reduced hospital readmissions, faster diagnoses, and lower overall healthcare costs. Examples of successful AI applications, such as AI-powered diagnostic tools for diseases like cancer, illustrate the potential ROI.
Comparative Cost Analysis of AI Healthcare Solutions
AI Healthcare Solution Category | Description | Estimated Cost Range (USD) | Justification for Cost |
---|---|---|---|
Diagnostics (e.g., image analysis) | AI systems for analyzing medical images (X-rays, MRIs, CT scans) to assist in diagnosis. | $10,000 – $1,000,000+ | Cost depends on the complexity of the algorithm, the required infrastructure, and the size of the image dataset. |
Treatment (e.g., personalized medicine) | AI systems to recommend personalized treatment plans based on patient data. | $50,000 – $5,000,000+ | Cost depends on the depth of patient data analysis, the sophistication of the recommendation algorithms, and the integration with existing systems. |
Drug Discovery | AI systems accelerating the drug discovery process. | $1,000,000 – $100,000,000+ | Cost is heavily influenced by the scale of the research, the sophistication of the algorithms, and the necessary infrastructure for laboratory work. |
Human Expertise Required
AI in healthcare, while promising, is not a plug-and-play solution. Successful implementation hinges critically on the human element, ensuring ethical use and optimal patient outcomes. This crucial aspect involves not only the development and implementation phases but also the ongoing maintenance and refinement of these complex systems. Expert human oversight is paramount to realizing the full potential of AI while mitigating potential risks.The development and application of AI in healthcare necessitate a deep understanding of medical processes, patient needs, and ethical considerations.
Human expertise is indispensable in translating medical knowledge into a format that AI can understand, ensuring accuracy and reliability in the output. Moreover, humans are vital in interpreting AI’s findings and ensuring appropriate clinical judgment and decision-making.
Essential Human Roles in AI Healthcare Development
The successful development and implementation of AI systems in healthcare require a diverse range of human expertise. These professionals act as critical links between the technical aspects of AI and the practical application within the clinical setting.
- Data Scientists and Engineers: These professionals are crucial in collecting, cleaning, and preparing the vast datasets needed to train AI models. Their expertise in data analysis, machine learning algorithms, and programming languages like Python is essential. They need to ensure the data is representative, unbiased, and of high quality, directly impacting the AI’s accuracy and reliability. Examples include transforming medical images, lab results, and patient records into usable data for the AI models.
- Medical Doctors and Clinicians: Their profound understanding of medical knowledge and patient care is essential in designing AI algorithms, validating their outputs, and ensuring their alignment with clinical best practices. They contribute to the creation of relevant datasets and provide crucial feedback on the system’s performance. For instance, cardiologists might help design AI models for heart disease diagnosis, ensuring that the model considers all relevant clinical factors.
- Biomedical Engineers: These professionals bridge the gap between medical science and engineering. Their role involves developing and testing the hardware and software components of AI systems. They ensure the systems are compatible with existing medical equipment and can integrate seamlessly into the healthcare workflow. Examples include designing sensors and devices for collecting physiological data or developing user interfaces for interacting with the AI.
Skills Needed for Each Role
The specific skills required for each role vary depending on the specific AI application. For example, a data scientist working on an AI model for predicting patient readmission might require more expertise in statistical modeling than a biomedical engineer developing a robotic surgery assistant.
- Data Scientists: Strong programming skills (Python, R), machine learning knowledge, data visualization, and statistical analysis expertise.
- Medical Doctors: Deep understanding of medical concepts, clinical practice, critical thinking, and communication skills.
- Biomedical Engineers: Proficiency in engineering principles, knowledge of medical devices, and the ability to integrate AI systems into existing healthcare workflows.
Comparison of Expertise Across AI Healthcare Applications
The required expertise varies based on the specific application. An AI system for diagnosing diabetic retinopathy requires a strong understanding of ophthalmology and image analysis, while an AI for personalized medicine requires expertise in genomics and pharmacogenomics.
Potential for Job Displacement and Retraining
While AI can automate certain tasks, it’s crucial to recognize that it’s not a replacement for human expertise. The potential for job displacement exists, but retraining and upskilling programs can prepare healthcare professionals for new roles that integrate AI. This could involve training in AI-assisted diagnostics, AI-driven personalized treatment plans, or roles focused on overseeing and interpreting AI outputs.
Human Roles in AI Healthcare Project Stages
Project Stage | Human Role | Training Requirements |
---|---|---|
Development | Data Scientists, Medical Doctors, Biomedical Engineers | Specialized courses in machine learning, medical knowledge, and engineering principles |
Implementation | Clinicians, IT Staff, Project Managers | Training on using the AI system, understanding its limitations, and integrating it into existing workflows |
Maintenance | Data Scientists, Medical Doctors, IT Staff | Continuous professional development in AI updates, medical advancements, and system maintenance |
Integration Challenges
The successful implementation of AI in healthcare hinges on its seamless integration with existing infrastructure and workflows. This process is not without its complexities, as disparate systems and varying data formats pose significant hurdles. Addressing these integration challenges is crucial for realizing the full potential of AI in improving patient care and operational efficiency.The integration of AI into healthcare systems is not a simple plug-and-play process.
It requires careful planning, significant technical expertise, and a thorough understanding of the existing infrastructure. Successfully navigating these challenges is paramount to realizing the benefits of AI-powered solutions in healthcare.
Obstacles in Integrating AI into Existing Healthcare Infrastructure
Existing healthcare infrastructure is often fragmented, with various systems and platforms developed over time, potentially using different technologies and data formats. This heterogeneity poses a major obstacle in integrating AI systems. Legacy systems may not be compatible with newer AI tools, necessitating costly upgrades or workarounds. Data silos, where information is stored in isolated systems, further complicate the integration process, making it difficult to access and utilize the necessary data for AI training and analysis.
This can result in inconsistent data quality and incomplete information, ultimately affecting the accuracy and reliability of AI-driven insights.
Data Privacy and Security Challenges Related to AI Systems
Healthcare data is highly sensitive, and maintaining its confidentiality is paramount. AI systems often rely on vast amounts of patient data, raising concerns about data breaches and unauthorized access. Robust security measures are essential to protect patient privacy and comply with regulations like HIPAA. Implementing strong encryption, access controls, and data anonymization techniques are critical components of any AI integration strategy.
Furthermore, ensuring compliance with data privacy regulations throughout the AI lifecycle, from data collection to analysis and disposal, is a crucial aspect of successful implementation.
Importance of Interoperability Between Different AI Systems and Existing Healthcare Software
Interoperability between AI systems and existing healthcare software is essential for seamless data exchange and efficient workflow integration. Different AI tools often use incompatible data formats, hindering their ability to communicate and share information. Standardized data formats and APIs are crucial to facilitate interoperability. Lack of interoperability can lead to redundant data entry, errors in data transmission, and inefficient workflows, ultimately impacting patient care.
This underscores the need for industry-wide collaboration and the development of common standards for data exchange.
Legal and Ethical Considerations for AI in Healthcare
The use of AI in healthcare raises important legal and ethical considerations. Issues such as algorithmic bias, accountability for AI-driven decisions, and the potential displacement of healthcare professionals need careful consideration. Transparency in AI algorithms is crucial to ensure fairness and prevent unintended consequences. Furthermore, ethical guidelines and regulations are necessary to address the unique challenges posed by AI in healthcare.
So, healthcare AI might seem like the future, but it’s a pricey one. You need highly skilled people to develop, maintain, and troubleshoot these systems. This often means needing top-notch hosting for your AI projects. Fortunately, finding reliable and affordable options like best cheap WordPress hosting can help offset some of the costs. Ultimately, while AI is promising, the human element remains critical and expensive in the healthcare sector.
Careful consideration of the ethical implications of AI deployment in clinical settings is crucial to avoid harm and maintain trust.
Necessity for Standardization in Data Formats for Seamless AI Integration
Standardization in data formats is essential for seamless integration of AI systems into existing healthcare infrastructure. Varied data formats across different systems can create significant obstacles. The use of standardized formats like FHIR (Fast Healthcare Interoperability Resources) ensures that data can be easily exchanged and utilized by various AI systems and healthcare applications. This approach minimizes the need for costly data transformations and promotes interoperability.
The adoption of standardized data formats can streamline the entire AI integration process, ultimately improving the efficiency and efficacy of healthcare operations.
Integration Challenges, Solutions, and Potential Impacts
Integration Challenge | Potential Solutions | Potential Impact on Healthcare Systems |
---|---|---|
Heterogeneous infrastructure | Modular design, cloud-based platforms, API integrations | Improved efficiency, reduced redundancy, streamlined workflows |
Data privacy and security | Strong encryption, access controls, anonymization techniques, compliance with regulations | Enhanced patient trust, protection of sensitive data, avoidance of data breaches |
Lack of interoperability | Standardized data formats (e.g., FHIR), common APIs, vendor collaboration | Seamless data exchange, efficient information flow, reduced errors |
Algorithmic bias | Diverse training datasets, regular audits, human oversight | Improved fairness and equity in AI-driven decisions, reduction of biases |
Standardization issues | Industry-wide collaboration, adoption of open standards, interoperability initiatives | Streamlined data exchange, reduced costs, improved data quality |
Human-AI Collaboration
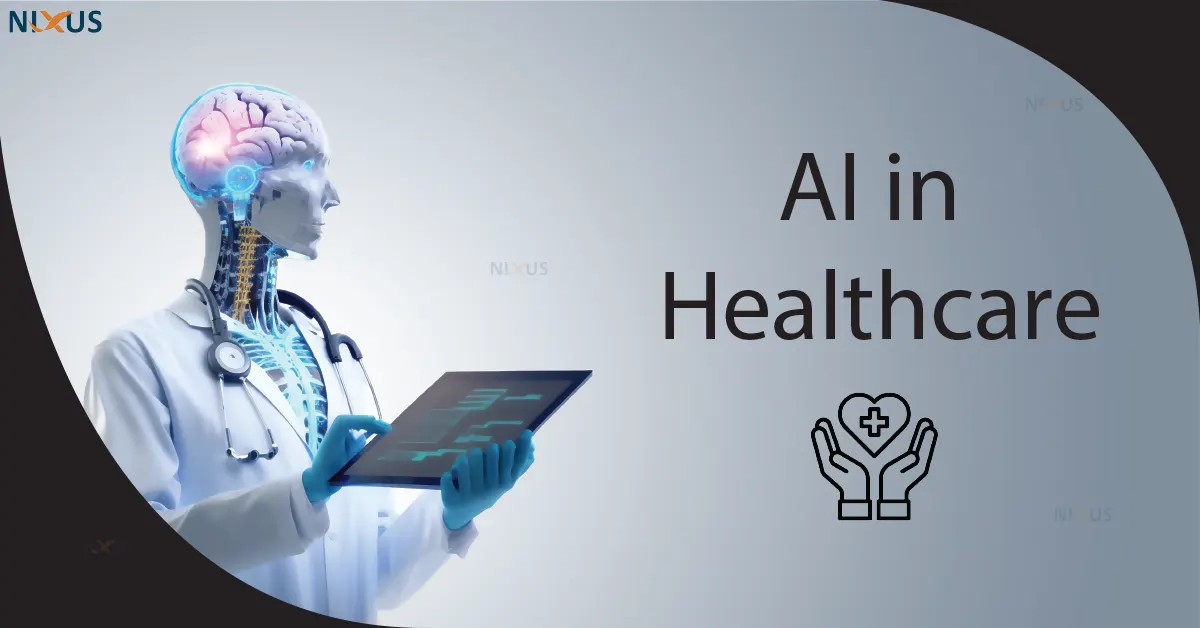
The integration of Artificial Intelligence (AI) into healthcare promises significant improvements in patient care, but it’s not a replacement for human expertise. Instead, a successful future hinges on a symbiotic relationship between AI and healthcare professionals. This collaborative approach leverages the strengths of both, enabling more efficient and effective diagnoses, treatments, and overall patient outcomes.AI’s ability to process vast amounts of data and identify patterns often surpasses human capabilities, while human clinicians possess crucial judgment, empathy, and nuanced understanding of individual patient needs.
The key lies in recognizing and utilizing these complementary strengths to create a system that enhances, rather than diminishes, the human touch in healthcare.
Examples of Successful Human-AI Collaborations
Several successful examples demonstrate the potential of human-AI partnerships in healthcare. One prominent example is the use of AI in radiology. AI-powered tools can analyze medical images like X-rays and CT scans, flagging potential anomalies that might be missed by human radiologists. This allows for faster diagnoses and potentially reduces the risk of misdiagnosis. Another area of success is in drug discovery, where AI algorithms can analyze vast datasets of molecular structures and identify potential drug candidates much faster than traditional methods.
Potential Benefits of Collaboration
Human-AI collaboration in healthcare offers numerous potential benefits. Increased diagnostic accuracy, quicker turnaround times for diagnoses, personalized treatment plans tailored to individual patient needs, and improved efficiency in administrative tasks are all potential outcomes. The ability to process vast amounts of data allows for more comprehensive patient assessments, leading to more effective treatment strategies. Furthermore, AI can automate routine tasks, freeing up healthcare professionals to focus on patient care and complex cases.
While AI might seem like the future of healthcare, the reality is that sophisticated AI systems need a lot of human expertise to develop and maintain. This often translates into higher costs for everyone involved. For a fascinating look into another area where human expertise and planning are key, check out this guide on growing your own cannabis: grow your own green the ultimate guide to planning and growing cannabis at home.
Ultimately, even in the realm of AI-powered healthcare, the human element remains expensive and crucial for success.
Augmenting Human Capabilities
AI can augment human capabilities in several ways. AI can analyze medical images more quickly and accurately, potentially reducing diagnostic errors. By automating data entry and administrative tasks, AI can free up healthcare professionals to spend more time with patients and focus on complex cases. AI can also provide clinicians with personalized insights, helping them to make more informed decisions about patient care.
Limitations of AI Systems and Human Oversight
Despite its potential, current AI systems have limitations. AI algorithms are trained on data, and if the data contains biases, the AI system will reflect those biases. Human oversight is crucial to ensure fairness and equity in healthcare. AI systems can also struggle with complex or ambiguous cases, requiring human intervention for proper interpretation. Furthermore, maintaining the integrity of patient data and ensuring patient privacy are paramount.
Training Healthcare Professionals on Using AI Tools
Effective use of AI tools requires proper training for healthcare professionals. This training should encompass both the technical aspects of using the tools and the ethical considerations involved. Healthcare professionals need to understand the capabilities and limitations of the AI tools, how to interpret the results, and when to seek human input. Continuous learning and updating are also critical to keep pace with the rapid advancements in AI technology.
While healthcare AI promises efficiency, the reality is it still requires expensive human oversight. Finding the best CS2 server hosting, for example, involves human expertise in setup and maintenance, much like the complex tasks needed for AI systems in healthcare. Ultimately, even with advanced AI, the need for skilled human professionals in healthcare remains high.
Responsibilities of Each Party
In a human-AI collaboration, both parties have distinct responsibilities. Healthcare professionals are responsible for understanding the AI tools, interpreting results, and ensuring ethical use. AI developers and implementers have a responsibility to build accurate, unbiased, and transparent AI systems. Clear guidelines and protocols are essential to ensure effective collaboration and avoid conflicts.
AI Assistance in Daily Healthcare Tasks
Healthcare Task | AI Assistance |
---|---|
Diagnostic Imaging Analysis | Automated detection of anomalies, providing second opinions, and faster reporting. |
Patient Data Management | Automated data entry, analysis of patient trends, and generation of personalized reports. |
Treatment Planning | Identifying optimal treatment options based on patient data and medical literature, suggesting personalized treatment plans. |
Administrative Tasks | Scheduling appointments, managing billing, and responding to patient inquiries. |
Drug Discovery | Accelerating drug discovery by identifying potential drug candidates and predicting their efficacy. |
Ethical Considerations
The integration of AI into healthcare promises significant advancements, but also raises complex ethical dilemmas. Careful consideration of these implications is crucial to ensure responsible and equitable deployment of AI systems in patient care. Ethical frameworks must guide the development and implementation of AI tools, addressing potential biases, safeguarding patient data, and ensuring fairness and transparency.The ethical considerations surrounding AI in healthcare extend beyond the technical aspects.
They delve into fundamental questions about human responsibility, patient autonomy, and the equitable distribution of resources. Addressing these concerns proactively will be essential for building trust and ensuring that AI benefits all members of society.
Patient Consent and Data Privacy
Ensuring patient autonomy and data security is paramount in AI-driven healthcare. Robust consent protocols are necessary, allowing patients to understand how their data will be used and ensuring they have the right to opt out or amend their consent at any time. These protocols should be transparent and easily accessible, using clear and concise language. Strict adherence to data privacy regulations, such as HIPAA in the US, is critical to prevent unauthorized access or misuse of sensitive patient information.
Data anonymization and encryption techniques should be implemented to protect patient confidentiality.
Potential Biases in AI Algorithms
AI algorithms are trained on data, and if that data reflects existing societal biases, the algorithms can perpetuate and even amplify these biases in their predictions and recommendations. For example, if a dataset used to train an AI system for diagnosing a particular disease disproportionately includes patients from one demographic group, the AI may be less accurate or effective for other groups.
Bias mitigation strategies include diverse and representative datasets, careful algorithm design, and ongoing monitoring and evaluation of the system’s performance across different patient populations. Techniques like fairness-aware machine learning can be employed to address and mitigate these biases.
Responsibility of Developers and Users
The responsibility for ensuring equitable access to AI-powered healthcare extends beyond developers to healthcare providers and policymakers. Developers must design algorithms that are accessible and understandable to diverse user groups. Healthcare providers must be trained on how to effectively use and interpret AI outputs in a way that is consistent with clinical best practices. Policymakers must ensure equitable access to AI technologies, preventing them from exacerbating existing health disparities.
Transparency and accountability are essential throughout the entire process, from data collection to algorithm development and deployment.
Potential for Exacerbating Health Disparities
AI systems, if not designed and implemented carefully, have the potential to exacerbate existing health disparities. If access to AI-powered tools is limited to certain socioeconomic groups or geographic locations, it could widen the gap in healthcare quality and outcomes. For instance, if a remote rural community lacks access to AI-assisted diagnostic tools, patients in that area may face delayed or less effective treatment compared to those in more technologically advanced areas.
Addressing these disparities requires targeted interventions and policies aimed at ensuring equitable access to AI technologies.
Key Ethical Principles for AI Use in Healthcare, Health care ai requires expensive humans
Principle | Description |
---|---|
Patient Autonomy | Respecting patient rights and choices regarding their health information and treatment. |
Beneficence | Promoting the well-being and best interests of patients. |
Non-Maleficence | Avoiding harm to patients. |
Justice | Ensuring fair and equitable access to AI-powered healthcare for all. |
Privacy | Protecting sensitive patient data and maintaining confidentiality. |
Transparency | Clearly communicating how AI systems work and their limitations. |
Future Trends
The journey of AI in healthcare is accelerating, promising transformative improvements in patient care. Emerging technologies are poised to revolutionize diagnostics, treatment, and preventative measures, pushing the boundaries of what’s possible. Understanding these trends is crucial for healthcare providers, researchers, and policymakers to harness the potential of AI while mitigating potential risks.The future of AI in healthcare is characterized by a growing integration of advanced technologies.
This integration is not simply about adding AI tools to existing workflows, but about fundamentally reshaping how healthcare is delivered, personalized, and approached proactively. AI is not meant to replace human expertise, but rather to augment it, creating a more efficient and effective healthcare ecosystem.
Potential Developments in AI Healthcare
AI’s application in healthcare is rapidly expanding, moving beyond simple diagnostic tools to encompass more complex processes. The development of sophisticated algorithms, machine learning models, and sophisticated data analysis techniques is driving this expansion. These advancements enable AI systems to analyze vast datasets, identify patterns, and make predictions with increasing accuracy and efficiency. This has significant implications for disease prevention, personalized treatment plans, and overall healthcare outcomes.
AI in Personalized Medicine
Personalized medicine is rapidly becoming a reality through AI. AI algorithms can analyze patient-specific data, including genetics, lifestyle, and medical history, to tailor treatment plans and predict potential health risks. This approach allows for earlier intervention and more effective therapies, ultimately improving patient outcomes. Examples include AI-powered tools that predict drug efficacy based on individual genetic profiles or that identify patients at high risk for developing certain diseases.
AI in Preventative Care
AI’s ability to analyze large datasets allows for the identification of patterns and trends that could predict health risks. This predictive capability can be used to implement proactive strategies to prevent diseases. AI-powered wearable devices and apps can track patient health data and alert healthcare providers to potential issues, enabling timely interventions and improving overall well-being. Early detection and intervention are crucial for preventing chronic diseases, improving health outcomes, and reducing healthcare costs in the long run.
AI in Diagnostics
AI is rapidly transforming diagnostic capabilities in healthcare. AI algorithms can analyze medical images (X-rays, CT scans, MRIs) with remarkable accuracy, assisting radiologists in detecting anomalies and providing faster diagnoses. This leads to quicker treatment and improved patient outcomes. Moreover, AI-powered tools can analyze complex data, such as patient symptoms, medical history, and lab results, to identify potential diseases earlier than traditional methods.
This proactive approach is crucial for improving patient outcomes and reducing healthcare costs.
Challenges and Opportunities
While AI offers significant opportunities for improving healthcare, challenges remain. Ensuring data privacy and security is paramount. The ethical implications of AI in healthcare must be carefully considered. Furthermore, the need for continuous training and upskilling of healthcare professionals to effectively integrate AI tools into their workflows is crucial. The opportunities are enormous, including the potential to reduce healthcare costs, improve diagnostic accuracy, and personalize treatment plans.
AI’s role in preventative care is expected to grow, leading to a healthier and more proactive population.
Human-AI Collaboration
The future of human-AI interaction in healthcare involves a collaborative approach. Humans bring critical judgment, empathy, and the ability to contextualize information, while AI excels at data analysis and pattern recognition. This synergistic partnership allows for more comprehensive and effective patient care. The successful integration of AI into healthcare necessitates a clear understanding of the roles of both human and AI in the decision-making process.
Future Trends Table
Trend | Impact on Healthcare |
---|---|
AI-powered diagnostics | Improved accuracy and speed of diagnosis, reduced errors, earlier interventions |
Personalized medicine | Tailored treatments, increased efficacy, improved patient outcomes |
Preventative care | Proactive disease management, early intervention, reduced healthcare costs |
AI-driven drug discovery | Faster development of new treatments, reduced research costs, more targeted therapies |
Remote patient monitoring | Enhanced accessibility, improved chronic disease management, reduced hospital readmissions |
Epilogue: Health Care Ai Requires Expensive Humans
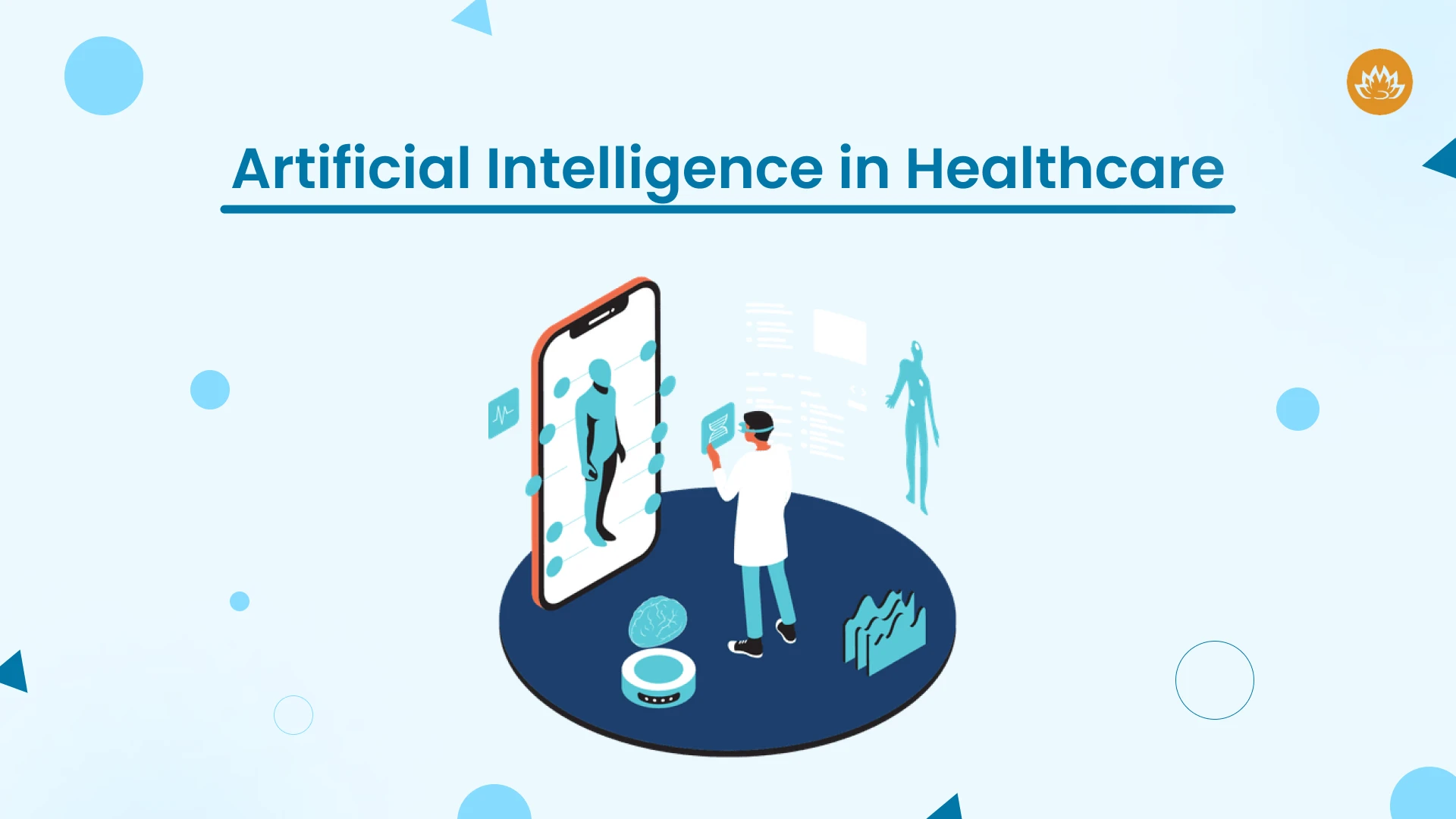
In conclusion, while AI holds immense promise for healthcare, the reality of its implementation is more nuanced. Beyond the alluring possibilities of AI, lies a complex web of financial, human, and ethical considerations. The integration of AI requires not only significant financial investment but also the expertise of skilled human professionals. This highlights the importance of careful planning and collaboration to ensure AI enhances, not replaces, the human element in healthcare.